Data Access and Data Insights for Everyone
Accelerate your business with data and AI
The MOSTLY AI Data Intelligence Platform
Access, create, and analyze data seamlessly using the AI Assistant — unlock insights that power innovation
Get started
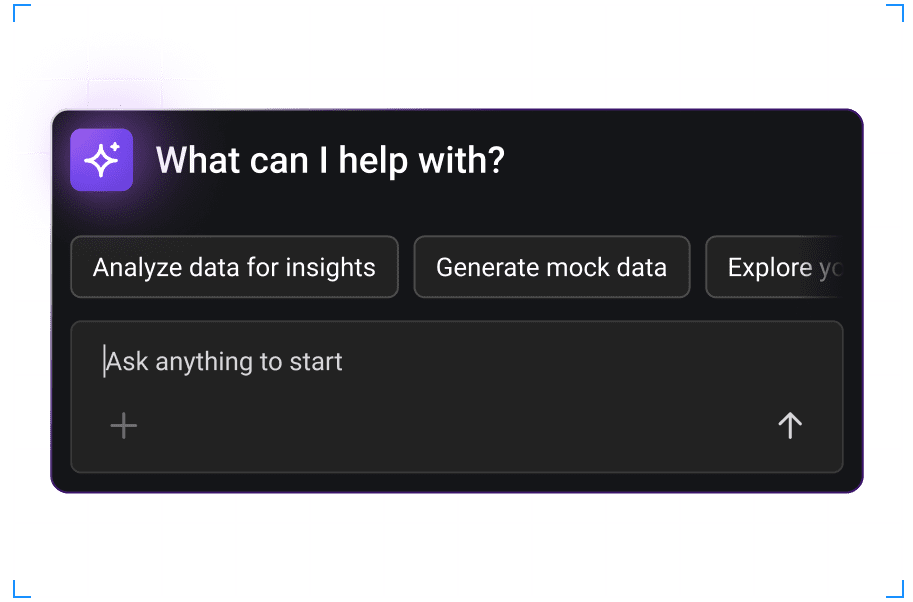
The Open Source Synthetic Data SDK
Create synthetic data locally in your Python environment — no sign-up, no need to upload data anywhere
Star on GitHub
Powering the world’s best data teams
The MOSTLY AI Data Intelligence Platform
Unlock the power of data
Access and work with production data securely, generate high-quality, privacy-safe synthetic data, and seamlessly analyze and share data across teams. With agentic data science at its core, the Platform enables organizations to accelerate AI innovation, streamline workflows, and drive smarter decision-making at scale.
Agentic. Secure.
For everyone.
Built for the Enterprise. Connect to your data within your secure environment. Run on your compute. Gain insights from your production data with the AI Assistant. Leverage synthetic data to broaden data access across your whole organisation.
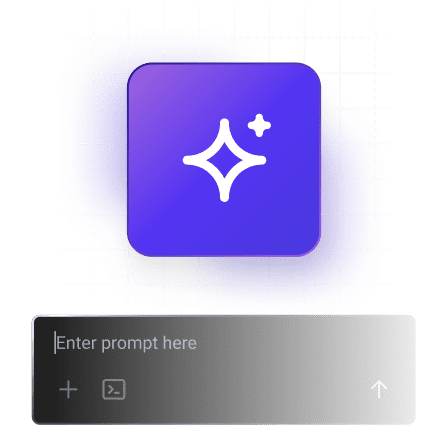
AI-powered insights
Use simple natural language, to run Python code and analyze your data.
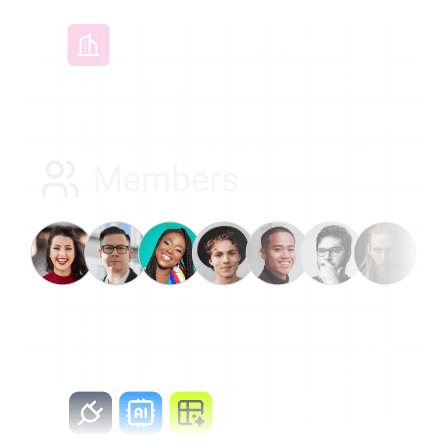
Teamwork made easy
Organize, manage, and collaborate on shared assets with your team.
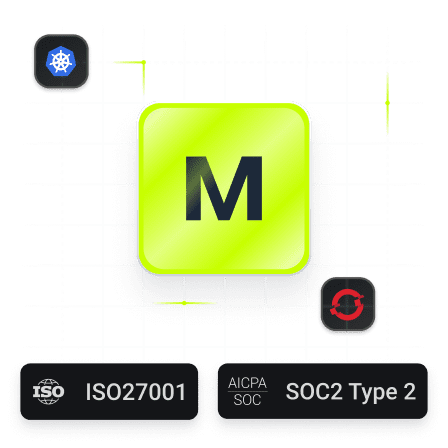
Enterprise-ready
Scalable, secure deployment on Kubernetes, OpenShift, or a VM.
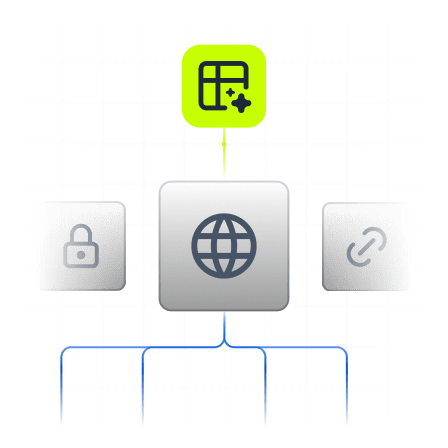
Share data globally
Create privacy-safe synthetic data and share it with the world.
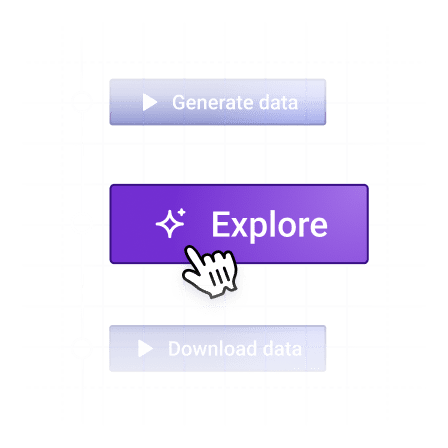
Simple & powerful
An easy-to-use platform for everyone, from beginner to expert.
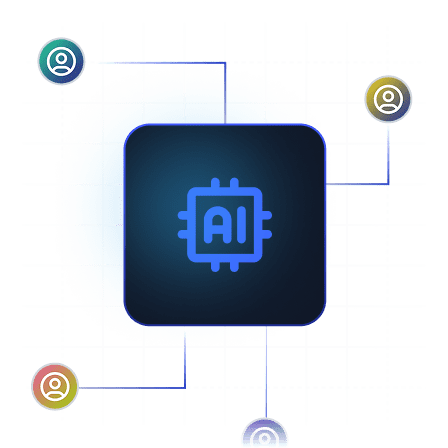
Built for AI
Accelerate your AI workloads by creating the data your teams need.
The Synthetic Data SDK
Get started instantly
Powered by the industry leading TabularARGN model architecture, generate high-fidelity synthetic data with built-in differential privacy, 100x faster training, advanced sampling, and support for complex tabular and textual datasets.
A fully permissive Open Source project under an Apache v2 license.
Learn moreA fully permissive Open Source project under an Apache v2 license.
!pip install -U mostlyai
# initialize the SDK
from mostlyai.sdk import MostlyAI
mostly = MostlyAI()
# train a generator
g = mostly.train(data="/path/to/data")
# inspect generator quality
g.reports(display=True)
# generate any number of new privacy-safe samples
mostly.probe(g, size=1_000_000)
# generate new synthetic samples to your needs
mostly.probe(g, seed=[{'age': 65, 'gender': 'male'}])
# export and share your generator
g.export_to_file()
Copied
Your data never leaves your environment
Create synthetic data locally in your Python environment - you stay in full control of your data.
Seamless integration
Export your Generators and upload them to the MOSTLY AI Data Intelligence Platform for exploration and sharing.
Synthetic data. Real results.