- What is data migration?
- How to do data migration with synthetic data?
- Challenges in data migration
- The role and benefits of synthetic data generation in data migration
- Real-world use cases and scenarios
- Real data migration vs synthetic data migration
- How to do data migration? Use synthetic data!
What is data migration, and how to do it well
Data migration is a critical process in data management and technology. Whether it involves upgrading systems, transitioning to the cloud, or consolidating databases, data migration is an integral part of keeping an organization's data infrastructure up-to-date and efficient.
However, data migration comes with its own set of challenges. Ensuring data integrity, preserving data privacy, and validating the accuracy of transferred data are among the primary concerns of data professionals. This is where synthetic data steps in as a powerful and innovative solution.
Synthetic data is artificial data that mimics the characteristics of real data without containing any actual information about individuals or entities. It's generated through mathematical models, algorithms, or statistical techniques. In the context of data migration projects, synthetic data serves as a solution, offering a host of benefits that address the challenges associated with traditional data migration.
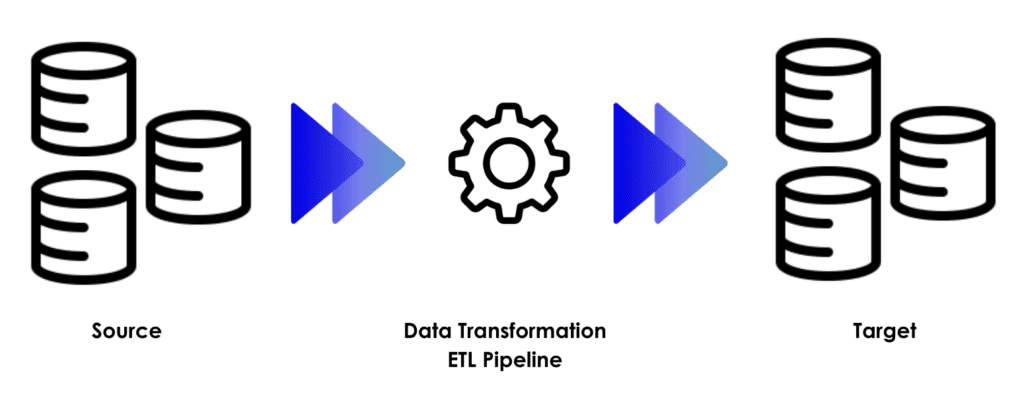
How to do data migration with synthetic data
So, why is synthetic data relevant in the context of data migration projects?
Imagine this scenario: You're tasked with migrating a massive customer database from one system to another. The data contains sensitive information like names, addresses, and purchase histories. It is critical to ensure data privacy and security. You must also extensively test your migration process to avoid data damage, loss, or format discrepancies. Furthermore, the enormous amount of data makes testing difficult and time-consuming.
This is precisely where AI-generated synthetic data shines. It allows you to create data that looks, feels, and behaves like the real thing, without exposing sensitive information. Synthetic data provides a controlled and secure environment for testing, ensuring that the migration process works seamlessly while safeguarding privacy and security.
In the following sections, we'll explore in detail how synthetic data addresses these challenges and offers a pathway to smoother, more efficient, and privacy-conscious data migration. We'll discuss its benefits, use cases, best practices, and more, so you can leverage the power of synthetic data to enhance your data migration projects.
Challenges in data migration
Data migration is a multifaceted process that, while necessary, often presents several challenges and complexities. These challenges can vary depending on the scale, scope, and nature of the migration project.
- Data Format Discrepancies: One of the most difficult aspects of data migration is dealing with data format discrepancies between the source and target systems. These distinctions can include differences in data architecture, data formats, and encoding standards. It is a difficult process to migrate data while verifying that it complies with the new format.
- Data Cleansing and Quality Assurance: Data quality concerns are widespread, and they may worsen during data transfer. Data that is inaccurate, incomplete, or duplicate might cause mistakes and inconsistencies in the target system. Cleaning and verifying data to ensure it meets quality requirements takes time and attention to detail.
- Data Volume and Scale: Large data migrations may strain resources and infrastructure. To prevent performance bottlenecks, data migration initiatives must be prepared to handle huge datasets efficiently. This frequently necessitates meticulous planning and optimization.
- Data Mapping and Transformation: Data is frequently mapped and converted to meet the requirements of the target system. This entails developing rules and logic for data conversion, which can be complex and error-prone if not handled methodically.
- Data Privacy and Security: It is critical to safeguard sensitive data during relocation. It is difficult to ensure that sensitive information is not exposed or compromised, especially when dealing with legislation such as GDPR or HIPAA.
- Testing and Validation: Testing and validation are required to ensure that the migration process is error-free and that the data in the target system appropriately replicates the source data. This entails writing test cases and ensuring that they cover a wide range of situations and edge scenarios.
Organizations frequently seek new ways to expedite the data migration process in consideration of these challenges. One such solution is the integration of synthetic data using MOSTLY AI’s synthetic data generator, which can significantly mitigate many of these challenges by providing a controlled, secure, and privacy-conscious environment for testing and validation without exposing sensitive information. If this piques your interest, please read on to understand how MOSTLY AI addresses these challenges and contributes to successful data migration projects.
The role and benefits of synthetic data generation in data migration
As explained above, data migration often involves transferring data from one system to another, and these systems may have different data formats, structures, and schemas. These format disparities can pose significant challenges, as data from the source system may not align neatly with the requirements of the target system.
Synthetic data generation excels in the task of harmonizing data formats by being adaptable and customizable. Synthetic data generators like MOSTLY AI, are designed to replicate the structure and format of the target system. This means that when you generate synthetic data for testing, it can match the schema and format specifications of the system you're migrating data to. When you test the migration process using synthetic data that matches the target system's format, you reduce the risk of errors and unexpected issues during the actual migration. This alignment ensures a smoother transition, as data is more likely to fit seamlessly into the new system without the need for complex data transformations or extensive manual adjustments.
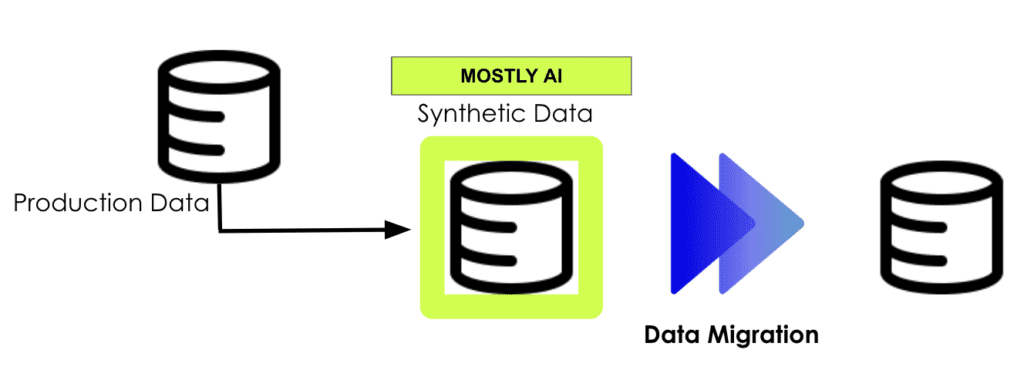
Data professionals during their data migration journey, can realise that synthetic data generated by MOSTLY AI plays a crucial role in maintaining data integrity and streamlining testing processes. Data integrity is protected via synthetic data. Its well-defined characteristics make any inconsistencies or errors during migration immediately noticeable. This allows for quick identification and resolution of issues, ensuring the accuracy and reliability of the migrated data.
On top of that, synthetic data simplifies the testing of data mapping and transformation rules. Organizations can create diverse test scenarios that encompass various mapping and transformation scenarios. This approach ensures comprehensive testing and validation, reducing the risk of errors in the migration process.
Synthetic data stands as a solution for organizations seeking to maintain data privacy and security during testing and validation. Its artificial nature, compliance with privacy regulations, high level of security, and customization options make it an ideal choice, especially in scenarios where real-world sensitive information must be shielded from exposure and potential breaches. MOSTLY AI is a well-known solution for protecting personally identifiable information (PII), maintaining data privacy and security of your original data.
Real-world use cases and scenarios
Data migration in insurance
Scenario: An insurance company is migrating policyholder data from legacy systems to a modern platform to enhance customer service and streamline operations.
Use of Synthetic Data: Synthetic policyholder profiles can be generated to replicate real policy data, including policy types, coverage details, and claims history. This synthetic data allows thorough testing of the migration process, ensuring data accuracy, privacy compliance, and adherence to insurance industry regulations.
Data migration in healthcare
Scenario: A healthcare provider is transitioning to a new electronic health record (EHR) system, necessitating the transfer of patient medical records.
Use of Synthetic Data: Synthetic patient records can be created to simulate real EHRs, preserving patient privacy and complying with strict healthcare regulations like HIPAA. These synthetic records enable comprehensive testing and validation of the EHR migration process, including data mapping and access control.
Data migration in financial services and banking
Scenario: A financial institution is merging with another bank, requiring the consolidation of customer accounts, transaction histories, and investment portfolios.
Use of Synthetic Data: Synthetic customer profiles and financial transactions can be generated to replicate real banking data. This synthetic data facilitates rigorous testing and validation of the migration, ensuring data accuracy, compliance with financial regulations, and the security of sensitive financial information.
Real data migration vs synthetic data migration
Differences | Real Data Migration | Synthetic Data Migration |
Data Privacy and Compliance | Using real data in testing poses privacy risks, requiring extensive measures to anonymize or pseudonymize sensitive information. Compliance can be challenging to maintain. | Synthetic data is artificial and does not contain real-world information, making it ideal for testing without privacy concerns. It ensures compliance with data protection regulations. |
Data Security | Handling real data in testing can expose sensitive information to security risks, necessitating stringent security measures. | Synthetic data is generated in controlled environments and is devoid of real-world vulnerabilities, making it highly secure. |
Testing Scenarios | Testing with real data may be limited by the availability of specific scenarios or edge cases, potentially leaving gaps in validation. | Synthetic data allows for the creation of diverse and extreme testing scenarios, ensuring comprehensive validation of migration processes. |
Scalability | Testing with massive datasets can be resource-intensive and challenging to manage. | Synthetic data can be generated at any scale, making it suitable for testing large-scale migrations. |
Flexibility | Real data may require complex transformations to align with the target system, increasing the risk of errors. | Synthetic data can be customized to match target system formats, facilitating format harmonization. |
How do you do data migration? Use synthetic data!
In the world of data migration, synthetic data from MOSTLY AI emerges as the game-changing solution. It simplifies processes, enhances privacy, and ensures data accuracy in sectors like insurance, healthcare, and finance.
Explore MOSTLY AI's synthetic data generator for seamless data migration. Discover how synthetic data can boost your organization's data migration projects while ensuring data privacy and compliance.