Access to healthcare data is crucial for conducting research on machine learning applications for the medical domain, particularly for developing models that can effectively address critical healthcare questions. For instance, Survival Analysis (SA) models trained on clinical trial data can predict patient survival times, disease recurrence, or responses to specific treatments, significantly informing clinical decision-making. However, due to privacy concerns and regulatory restrictions, access to sensitive patient data is often limited.
One potential solution to this challenge is the use of synthetic data, which closely mimics real-world datasets. When coupled with privacy-preserving mechanisms, synthetic versions of clinical datasets can enable researchers and healthcare practitioners to build, test, and validate a variety of models securely and ethically. In a broader sense, synthetic data represents an important step toward democratizing datasets, potentially granting researchers and practitioners access to information otherwise restricted due to ethical concerns.
In this post, we’ll illustrate how synthetic data can support and enhance Survival Analysis (SA), a critical tool in healthcare for predicting time-to-event outcomes such as patient mortality, disease progression, or hospital readmission. We’ll leverage the popular pycox and mostlyai packages to demonstrate this approach. You find a notebook with reproducible code here.
Understanding Survival Analysis and the Cox Model
Survival Analysis (SA) is a statistical framework that models the time until an event of interest occurs. In the context of healthcare, one could be interested in modeling health outcomes such as patient mortality, disease recurrence, or hospital readmission. A central concept in SA is the hazard function, which describes the instantaneous risk or rate at which the event occurs at a specific point in time, given that the event hasn’t yet occured.
The Cox Proportional Hazards Model is a widely used method in SA, which estimates the hazard function as follows:
h(t|x) = h0(t)exp (fϕ(x))
where:
- h(t|x) is the hazard function at time t, given covariates x
- h0(t) is the baseline hazard function
- fϕ(x) represents the effect of covariates, and is a function than can be parameterized by a neural network.
The proportional hazards property of the Cox model assumes that the relationship between covariates and the event risk remains consistent over time. In other words, while the absolute risk (baseline hazard) can vary, the relative risk between subjects — captured by their covariates through exp (fϕ(x)) - stays constant. The Cox model leverages the partial likelihood function during training, allowing it to estimate covariate effects without explicitly modeling the underlying baseline hazard. This makes the model versatile and highly effective for various survival prediction tasks.
Why Use Synthetic Data for Survival Analysis?
As discussed earlier, healthcare applications frequently face challenges due to limited access to real patient data, and Survival Analysis (SA) is no exception. However, what if we had a dataset that closely mimics real-world patient data without privacy concerns? Synthetic data kicks in to offer a practical solution to this problem. Generated with privacy-preserving methods, synthetic data enables researchers to train and validate SA models effectively while safeguarding patient privacy.
In what follows we aim at demonstrating precisely that: the value that private synthetic data can bring into SA when the real patient data is not readily accessible. For the purpose of this demonstration we utilize the SUPPORT dataset, a real-world and publicly available survival dataset comprising critically ill patients in Intensive Care Units (ICUs). This dataset includes time-to-event information, clinical and demographic features, and indicators of specific events (such as patient mortality).
Synthetic Data Generation and Survival Model Training
To demonstrate the practical value of synthetic data for Survival Analysis (SA), we’ll follow a straightforward experimental pipeline. Our goal is to show that models trained on privacy-preserving synthetic data can perform comparably to models trained directly on real patient data. If successful, this suggests that synthetic data can be a useful alternative for developing survival models, balancing predictive performance with patient privacy considerations.
To do that, we’ll adopt the Train Synthetic Test Real (TSTR) framework to evaluate how effectively a Cox survival model, trained on synthetic data, can perform on a real-world holdout dataset. Specifically, we’ll train separate models on the original and synthetic datasets and then assess their performance on the true test set. Comparable performance between these models would indicate that the synthetic dataset is indeed valuable for this downstream survival task. The diagram below depicts the workflow of TSTR.
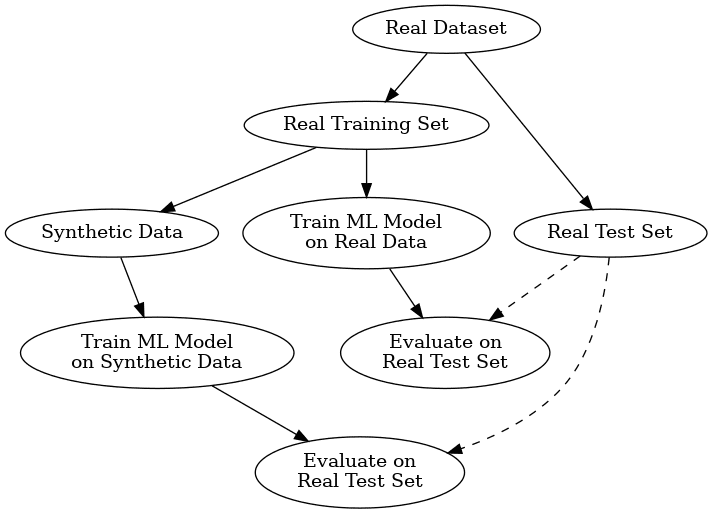
In what follows we’ll then generate a synthetic version of the training set, ensuring it closely mirrors the statistical properties of the original data. There’s a variety of privacy-preserving mechanisms available in the Synthetic Data SDK (you can read more about these here). In this blog we use a simple approach based on rare category protection, which masks rare categories and extreme values to decrease the risk of re-identification.
Generating Synthetic Survival Data
To create our synthetic dataset, we use the MOSTLY AI's Synthetic Data SDK. After having loaded and split the dataset, training a generator with the SDK is quite straightforward:
from pycox.datasets import support
# Load data
df = support.read_df()
df_test = df.sample(frac=0.2)
df_train = df.drop(df_test.index)
# Train generator
mostly = MostlyAI()
g = mostly.train(
config={
"name": "Support",
"tables": [
{
"name": "support",
"data": df_train,
"tabular_model_configuration": { # tabular model configuration (optional)
"max_training_time": 10,
"enable_flexible_generation": False,
"value_protection": True,
"model": "MOSTLY_AI/Medium",
"batch_size": 128,
},
"columns": [{'name': c, 'model_encoding_type': 'TABULAR_NUMERIC_DIGIT'} for c in df_train.columns],
}
],
},
start=True,
wait=True,
)
In the config
attribute we define the model configuration, including number of epochs, maximum training time, the column datatypes, etc. In particular, we set value_protection = True
to enable rare category protection, and we set the column encoding types to TABULAR_NUMERIC_DIGIT
. You can check how to fulfill the configuration here. Once trained, we can generate synthetic data as follows:
sd = mostly.generate(g, size=df_train.shape[0])
df_synthetic = sd.data()
A crucial aspect of SA is the relationship between the duration and the event occurrence: a synthetic dataset must preserve this relationship. Otherwise, a survival model that fits well the synthetic data might perform poorly when estimating the survival outcomes in the true holdout set. To make sure our synthetic dataset captures this relationship, let’s compare the distribution of durations grouped by event occurrence:
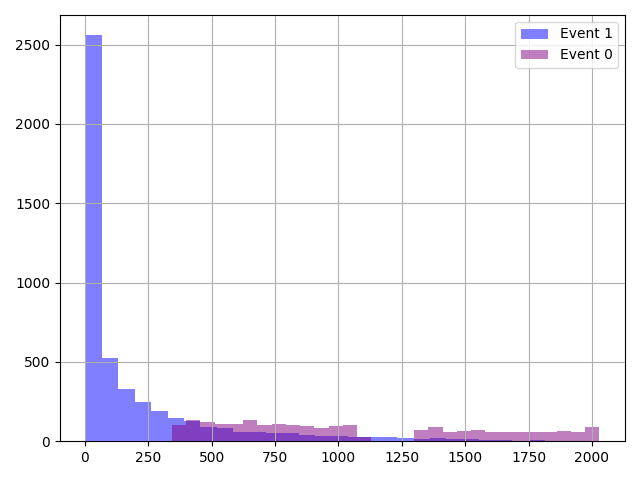
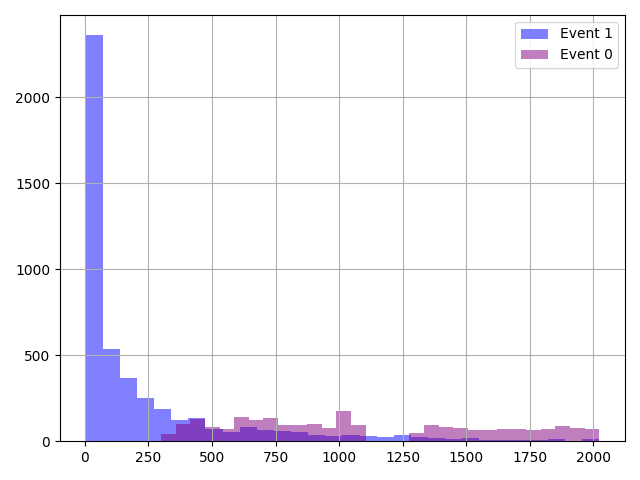
Distribution of durations grouped by event occurrence for the true training set (left) and synthetic training set (right).
As we can see, the synthetic dataset does a great job at emulating the distribution of durations and events. This is great news! Let’s now move forward to training survival models on the synthetic and real datasets.
Training a survival Cox model on the real and synthetic datasets
To train our Cox model, we first set aside 20% of the records from the training set to do survival model selection. We then apply standardization to both the training and validation splits. We parameterize the model with a two-layers MLP with ReLU activations, batch norm and dropout regularization. We use the MLPVanillaCoxTime
class from the pycox
to define the network architecture and the CoxTime
class to fit a Cox survival model. We set the learning rate to 0.01 and run until early stopping is triggered or for a maximum of 512 epochs. We use this exact procedure for both the real and synthetic datasets.
Model evaluation and results
To assess the quality of the models we use to popular metrics to evaluate the goodness of fit of survival models. These are the Concordance Index (CI) and the Integrated Brier Score (IBS). The CI measures how well the survival model ranks the subjects according to their predicted duration. A score closer to 1 represents a very accurate ranking. The IBS can be deemed an error score representing the average square distance between the observed survival outcome and the predicted survival probability across time steps. An IBS closer to 0 indicates the model fitted the data accurately.
To compute these metrics, we first run our trained models (both real-data-trained and synthetic-data-trained) on the true test set to estimate the survival outcomes for each patient. From these predictions, we then calculate CI and IBS, giving us a direct comparison of how well models trained on synthetic versus real data generalize to real-world scenarios. We present these results below:
Training Data | Concordance Index (CI) ↑ | Integrated Brier Score (IBS) ↓ |
---|---|---|
Real dataset | 0.575 | 0.204 |
Synthetic dataset | 0.593 | 0.199 |
These results indicate that the model trained on synthetic data is comparable with the model trained on real data directly. This supports the fact that privacy-preserving synthetic data can be a viable alternative for Survival Analysis in healthcare.
Conclusion
This experiment illustrates a simple yet promising use case for synthetic data in Survival Analysis for healthcare. We’ve shown that privacy-preserving synthetic data can serve as a practical substitute for real data. These results highlight its potential as a valuable alternative in scenarios where access to real patient data is limited, thus enabling researchers and practitioners to continue developing meaningful applications without compromising privacy.