The European Union’s Artificial Intelligence Act (“AI Act”) is likely to have a profound impact on the development and utilization of artificial intelligence systems. Anonymization, particularly in the form of synthetic data, will play a pivotal role in establishing AI compliance and addressing the myriad challenges posed by the widespread use of AI systems.
This blog post offers an introductory overview of the primary features and objectives of the draft EU AI Act. It also elaborates on the indispensable role of synthetic data in ensuring AI compliance with data management obligations, especially for high-risk AI systems.
Notably, we focus on the European Parliament’s report on the proposal for a regulation of the European Parliament and the Council, which lays down harmonized rules on Artificial Intelligence (the Artificial Intelligence Act) and amends certain Union Legislative Acts (COM(2021)0206 – C9-0146/2021 – 2021/0106(COD)). It's important to note that this isn't the final text of the AI Act.
The first comprehensive regulation for AI compliance
The draft AI Act is a hotly debated legal initiative that will apply to providers and deployers of AI systems, among others. Remarkably, it is set to become the world's first comprehensive mandatory legal framework for AI. This will directly impact researchers, developers, businesses, and citizens involved in or affected by AI. AI compliance is a brand new domain that will transform the way companies manage their data.
The choice: AI compliance or costly consequences
Much like the GDPR, failure to comply with the AI Act's obligations can have substantial financial repercussions: maximum fines for non-compliance under the draft AI Act can be nearly double those under the GDPR. Depending on the severity and duration of the violation, penalties can range from warnings to fines of up to 7% of the offender's annual worldwide turnover.
Additionally, national authorities can order the withdrawal or recall of non-compliant AI systems from the market or impose temporary or permanent bans on their use. AI compliance is set to become a serious financial issue for companies doing business in the EU.
Risk-based classification
The draft EU AI Act operates on the principle that the level of regulation should align with the level of risk posed by an AI system. It categorizes AI systems into four risk categories:
- Unacceptable risk: This pertains to AI applications that are prohibited due to violations of human dignity, democracy, or fundamental rights, such as social scoring systems or real-time biometric identification in public spaces. AI systems in this category are entirely banned.
- High risk: High-risk AI applications are subject to stringent obligations before they can be placed on the market or put into service. This includes AI used in critical infrastructure, education, employment, health, justice, or law enforcement.
- Limited risk: AI applications in this category, such as chatbots, must adhere to transparency requirements.
- Minimal risk: AI applications with minimal risk face no specific obligations, such as AI used for entertainment or personal purposes.
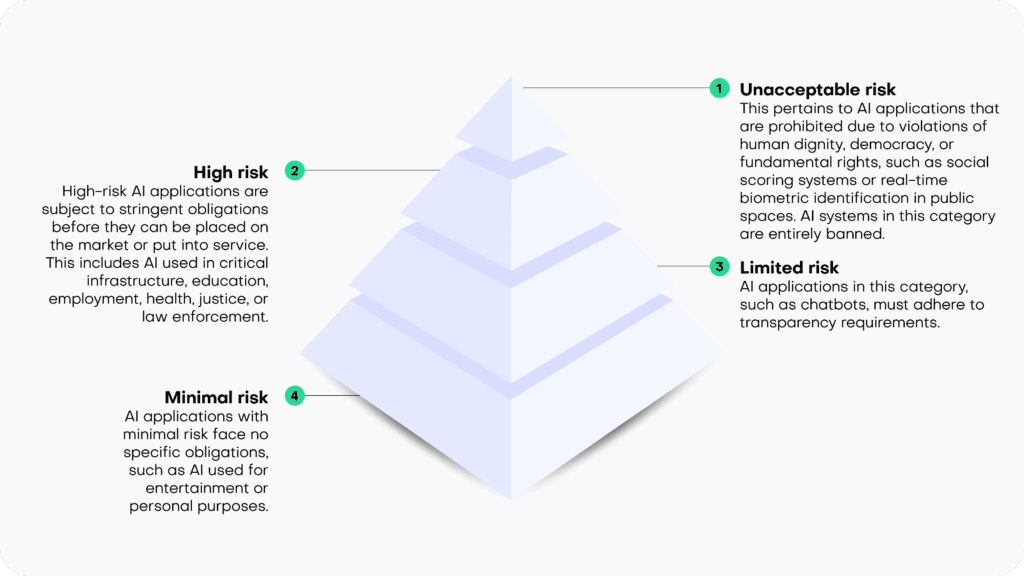
Synthetic solutions for AI compliance
The draft AI Act focuses its regulatory efforts on high-risk AI systems, imposing numerous obligations on them. These obligations encompass ensuring the robustness, security, and accuracy of AI systems. It also mandates the ability to correct or deactivate the system in case of errors or risks, as well as implementing human oversight and intervention mechanisms to prevent or mitigate harm or adverse impacts, as well as a number of additional requirements.
Specifically, under the heading “Data and data governance”, Art. 10 sets out strict quality criteria for training, validation and testing data sets (“data sets”) used as a basis for the development of “[h]igh-risk AI systems which make use of techniques involving the training of models with data” (which likely encompasses most high-risk AI systems).
According to Art 10(2), the respective data sets shall be subject to appropriate data governance and management practices. This includes, among other things, an examination of possible biases that are likely to affect the health and safety of persons, negatively impact fundamental rights, or lead to discrimination (especially with regard to feedback loops), and requires the application of appropriate measures to detect, prevent, and mitigate possible biases. Not surprisingly, AI compliance will start with the underlying data.
Pursuant to Art 10 (3), data sets shall be “relevant, sufficiently representative, appropriately vetted for errors and as complete as possible in view of the intended purpose” and shall “have the appropriate statistical properties […]“.
Art 10(5) specifically stands out in the data governance context, as it contains a legal basis for the processing of sensitive data, as protected, among other provisions, by Art 9(1) GDPR: Art 10(5) entitles high-risk AI system providers, to the extent that is strictly necessary for the purposes of ensuring negative bias detection and correction, to exceptionally process sensitive personal data. However, such data processing must be subject to “appropriate safeguards for the fundamental rights and freedoms of natural persons, including technical limitations on the re-use and use of state-of-the-art security and privacy-preserving [measures]“.
Art 10(5)(a-g) sets out specific conditions which are prerequisites for the processing of sensitive data in this context. The very first condition, as stipulated in Art 10(5)(a) sets the scene: the data processing under Art 10 is only allowed if its goal, namely bias detection and correction “cannot be effectively fulfilled by processing synthetic or anonymised data”. Conversely, if an AI system provider is able detect and correct bias by using synthetic or anonymized data, it is required to do so and cannot rely on other “appropriate safeguards”.
The distinction between synthetic and anonymized data in the parliamentary draft of the AI Act is somewhat confusing, since considering the provision’s purpose, arguably only anonymized synthetic data qualifies as preferred method for tackling data bias. However, since anonymized synthetic data is a sub-category of anonymized data, the differentiation between those two terms is meaningless, unless the EU legislator attempts to highlight synthetic data as the preferred version of anonymized data (in which case the text of the provision should arguably read “synthetic or other forms of anonymized data”).
Irrespective of such details, it is clear that the EU legislator clearly requires the use of anonymized data for the processing of sensitive data as a primary bias detection and correction tool. It looks like AI compliance cannot be achieved without effective and AI-friendly data anonymization tools.
Recital 45(a) supports this (and extends the synthetic data use case to privacy protection and also addresses AI-system users, instead of only AI system providers):
“The right to privacy and to protection of personal data must be guaranteed throughout the entire lifecycle of the AI system. In this regard, the principles of data minimization and data protection by design and by default, as set out in Union data protection law, are essential when the processing of data involves significant risks to the fundamental rights of individuals.
Providers and users of AI systems should implement state-of-the-art technical and organizational measures in order to protect those rights. Such measures should include not only anonymization and encryption, but also the use of increasingly available technology that permits algorithms to be brought to the data and allows valuable insights to be derived without the transmission between parties or unnecessary copying of the raw or structured data themselves.”
The inclusion of synthetic data in the draft AI Act is a continuation of the ever-growing political awareness of the technology’s potential. This is underlined by a recent statement made by the EU Commission’s Joint Research Committee: “[Synthetic data] not only can be shared freely, but also can help rebalance under-represented classes in research studies via oversampling, making it the perfect input into machine learning and AI models."
Synthetic data is set to become one of the cornerstones of AI compliance in the very near future.