According to Gartner, "data and analytics leaders who share data externally generate three times more measurable economic benefit than those who do not." Yet, organizations even struggle to collaborate on data within their own walls. No matter the architecture, somehow, everyone ends up with rigid silos and uncooperative departments. Why? Because data collaboration is a lot of work.
The data mesh approach to collaboration
Treating data as a product and assigning ownership to people closest to the origins of the particular data stream makes perfect sense. The data mesh architecture attempts to reassign data ownership from a central focal point to decentralized data owners with domain knowledge embedded into teams across the entire organization. But the data mesh is yet to solve the cultural issues. What we see time and time again at large organizations is people becoming overly protective of the data they were entrusted to govern. Understandably so. The zero trust approach is easy to adopt in the world of data, where erring on the side of caution is justified. Data breaches are multimillion-dollar events, damaging reputations on all levels, from organizational to personal. Without trusted tools to automatically embed governance policies into data product development, data owners will always remain reluctant to share and collaborate, no matter the gains interconnecting data products offer.
The synthetic data mesh for data collaboration
Data ecosystems are already built with synthetic data, accelerating AI adoption in the most data-critical industries, such as finance. When talking about accelerating data science in finance, Jochen Papenbrock, Head of Financial Technology at NVIDIA said:
"Synthetic data is a key component for evaluating AI models, and it's also a key component of collaboration in the ecosystem. My personal belief is that as we see a strong growth of AI adoption, and we'll see a strong growth in the adoption of synthetic data at the same speed."
So making synthetic data generation tools readily available for data owners should be considered a critical component of the data mesh. Proactively synthesizing and serving data products across domains is the next step on your journey of weaving the data mesh and scaling data collaborations. Readily available synthetic data repositories create new, unexpected value for data consumers and the business.
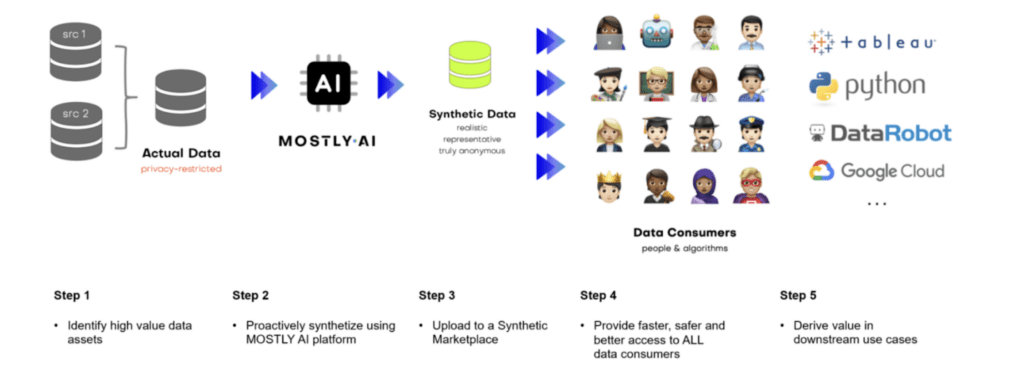
Examples of synthetic data products
Accelerating AI innovation is already happening at companies setting the standards for data collaborations. Humana, one of the largest North American health insurance providers, launched a synthetic data exchange to accelerate data-driven collaborations with third-party vendors and developers. Healthcare data platforms populated with realistic, granular and privacy safe synthetic patient data are mission-critical for accelerating research and product development.
Sometimes data silos are legal requirements, and certain data assets cannot be joined for compliance reasons. Synthetic data versions of these datasets serve as drop-in placements and can interconnect the domain knowledge contained in otherwise separated data silos. In these cases, synthetic data products are the only option for data collaboration.
In other cases, we've seen organizations with a global presence use synthetic data generation for massive HR analytics projects, connecting employee datasets from all over the world in a way that is compliant with the strictest regulations, including GDPR.
The wide adoption of AI-enabled data democratization represents breakthrough moments in how data consumers access data and create value. The intelligence data contains should no longer be locked away in carefully guarded vaults but flowing freely between teams and organizations.
The benefits of data collaborations powered by synthetic data
Shareable synthetic data helps data owners who want to collaborate and share data in and out of organizations by reducing time-to-data and governance costs, enabling innovation, democratizing data, and increasing data literacy. Unlike legacy data anonymization, which reduces data utility. The reduction in time-to-data in itself is significant.
"According to our estimates, creating synthetic data products results in a 90%+ reduction in time-to-consumption in downstream use cases. Less new ideas are left on the cutting room floor, and more data is making an impact in the business.” says John Sullivan, Head of Customer Experience at MOSTLY AI.
MOSTLY AI's synthetic data platform was created with synthetic data products in mind - synthetic data can be shared directly from the platform together with the automatically generated quality assurance report.
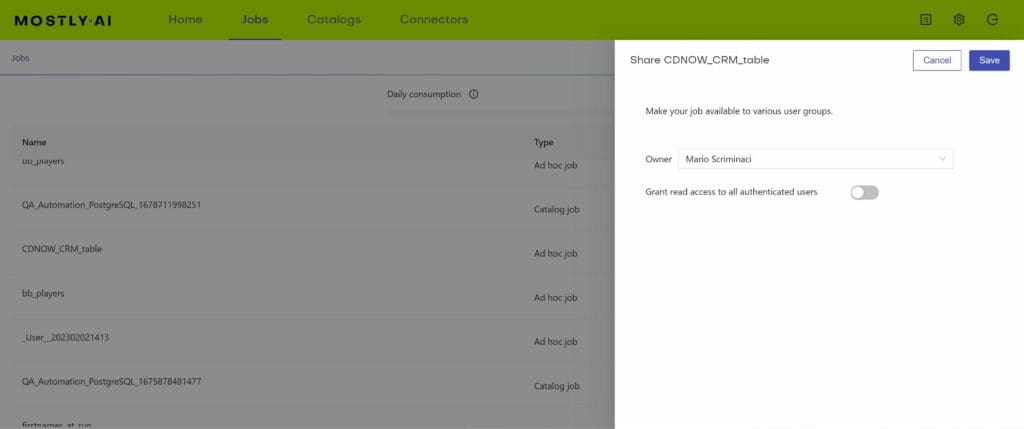
Data mesh vs. data fabric with synthetic data in mind
Mario Scriminaci, MOSTLY AI’s Chief Product Officer thinks, that the concept of the data mesh and data fabric is often perceived as antithetical.
“The difference between the two architectures is that the data mesh pushes for de-centralization, while the data fabric tries to aggregate all of the knowledge about metadata. In reality, they are not mutually exclusive. The concepts of the data mesh and the data fabric can be applied simultaneously in big organizations, where the complexity of data architecture calls for a harmonized view of data products. With the data consumption and data discovery initiatives, synthetic data generation will help centralize the knowledge of data and datasets (aka. the data fabric) and, at the same time, will also help customize datasets to domain-specific needs (aka. data mesh).”
In a data mesh architecture, data ownership and privacy are crucial considerations. Synthetic data generation techniques allow organizations to create realistic data that maintains privacy. It enables data collaboration between teams across organizations to produce and share synthetic data products with high utility.
Data mesh architectures promote the idea of domain-oriented, self-serve data teams. Synthetic data allows teams to experiment, develop, and test data pipelines and applications independently, fostering agility and making data democratization an everyday reality.
Synthetic data products also eliminate the need to replicate or move vast volumes of real data across systems, making it easier to scale and optimize data processing pipelines and enabling data collaboration at scale.