Table of Contents
The definition of data monetization
Data monetization refers to converting data assets into revenue or value for an organization. The strategic practice of data monetization involves the collection, analysis, and sale of data to generate profits, improve decision-making, or enhance customer experiences. We can distinguish between internal and external data monetization, depending on where data consumption takes place. Businesses can acquire a competitive advantage in the digital world by exploiting insights and information from data. Data monetization is critical in today's economy, allowing businesses to maximize the value of their data resources while adhering to privacy and security policies and regulations.
Motivation for data monetization: Data as an asset
The idea that data is an asset is becoming more and more prevalent, changing how businesses function and develop. Data is now more than simply a byproduct of operations; it's a product itself, a useful tool that can be used to improve decision-making, generate new income streams, and gain a competitive edge. This paradigm shift raises a fundamental question: why not include data as an asset on a company's balance sheet, just like other tangible and intangible assets?
The recognition of data as an asset is not a theoretical proposition but a practical reality. Companies have come to understand that the enormous amounts of data they generate, gather, and retain may contain a wealth of information about consumer preferences, market trends, and insights. By evaluating this data, organizations can improve operations, create more successful marketing campaigns, and make better decisions. The outcome? Increased effectiveness, financial savings, and — above all — the generation of fresh revenue streams.
Take online retail giants like Amazon and Netflix as an example. They have transformed their business models by utilizing data. They increase revenue and retention of customers by offering personalized suggestions based on an analysis of consumer behavior. They also generate extra revenue by selling insights to outside parties, which is another way they monetize data. They have been able to disrupt established markets and prosper in the digital age because of their data-centric strategy.
However, the potential of data as an asset extends far beyond these tech giants. All organizations, no matter how big or little, produce and gather data. Data is essential for improving goods and services, finding new market possibilities, and optimizing operations in a variety of industries, including manufacturing, financial services, and healthcare. Acknowledging data on balance sheets as an asset is a natural first step towards appreciating its actual value. This action may result in a more complete and transparent representation of a business's worth, giving stakeholders a greater understanding of its potential for expansion and data-driven capabilities.
How can synthetic data help?
When it comes to data monetization techniques, synthetic data can be a useful tool. It helps businesses maximize their data resources and opens up new revenue streams. How?
- Protecting sensitive information
By using synthetic data, businesses can profit from their data assets and get insightful knowledge without disclosing private or sensitive information. This is particularly important in sectors like healthcare and finance that are governed by stringent privacy laws, since actual data frequently contains sensitive or personal information. Legacy data anonymization tools can destroy data quality and decrease the value of data assets.
- Creating synthetic data marketplaces
It is possible to create data marketplaces using synthetic datasets, in which companies can provide insightful information to researchers, data scientists, and other enterprises. By selling or licensing these artificially generated datasets, new revenue streams may be generated.
- Enhancing data products
Synthetic data may be used by organizations that offer data services and products to improve and expand their product offerings. They can combine real and synthetic data to generate more comprehensive datasets that satisfy a wider range of customer expectations.
- Improve machine learning performance
Synthetic data offers a great upsampling method. It can be used in addition to real data to train machine learning models, increasing the models' accuracy and durability. This improved model performance can be quite helpful when offering predictive analytics services to clients.
- Risk mitigation
By using synthetic data for data monetization, the dangers of sharing real data can be reduced. Potential clients or partners may be more inclined to work together and invest in data-driven solutions as a result.
- Reducing data acquisition costs
Organizations can use synthetic data to address the needs of data-hungry applications and analytics while lowering expenses associated with data collection and storage, as opposed to continually collecting and storing real data.
- Enables data retention
Internal data retention policies and external legal requirements can make data retention impossible in the long run. However, the value of retaining data as an asset lies in its potential to provide historical insights, support trend analysis, and facilitate future decision-making. Retaining synthetic versions of datasets that must be deleted is a compliant and data-friendly solution, ensuring that the organizational knowledge base remains intact for strategic planning and informed decision-making while adhering to privacy and regulatory standards.
For the aforementioned reasons, synthetic data is an invaluable enabler that helps businesses maximize their data assets while resolving privacy issues and cutting expenses. It provides more assurance over data security and privacy compliance when entering into partnerships for data sharing, developing innovative data products, and expanding revenue streams.
Data monetization use cases
Synthetic data makes granular-level information accessible without requiring individual consent to share. This makes it possible for businesses to profit from their data assets while upholding data security and privacy, adhering to legal obligations, and making use of data-driven insights to improve decision-making and open up new revenue streams. This section will examine particular use cases from a variety of sectors, demonstrating how synthetic data can revolutionize data monetization by providing a competitive advantage and ensuring compliance with data protection laws.
- Data monetization in financial services
Financial institutions can use synthetic data in various ways — for example, to create credit scoring models and assess the risk of lending to individuals or businesses. By having access to granular-level information, they can make more informed decisions without violating data privacy regulations. Synthetic data can emulate the behavior of real customers without needing their consent. Banks can monetize this synthetic data by offering their advanced credit scoring and risk assessment models to other financial institutions or third-party vendors. These models, based on synthetic data, can provide valuable insights into creditworthiness and risk assessment. By licensing or selling these models, financial institutions can create an additional revenue stream while maintaining data privacy and security, making it a win-win scenario for both the bank and its partners.
- Marketing
Marketing firms can use synthetic data to segment customer profiles and create personalized marketing campaigns. This data can be shared with clients or partners without the need for individual customer consent. For example, banks can use synthetic data to optimize product recommendations for specific customer segments, creating personalized customer experiences and increasing customer satisfaction.
Marketing firms can monetize this valuable resource by offering their expertise in customer segmentation and their personalized marketing strategies based on synthetic data to other businesses in need of such services. By providing data-driven marketing solutions, marketing agencies can not only enhance their client offerings but also establish additional revenue streams through consulting and licensing agreements. This approach drives business growth and maintains the privacy and security of customer data, ensuring compliance with data protection regulations.
- Telecommunications
Telecommunications businesses can profit from synthetic data for network optimization. Network traffic and usage patterns can be simulated with synthetic data, which helps with infrastructure design and resource allocation. This may result in lower infrastructure costs and better service quality.
Telecommunication companies can generate money from this vital expertise, which is driven by synthetic data, by charging other service providers or companies looking to improve their network performance. Telecom firms can add value proposition products to their offerings and diversify their revenue streams by delivering data-driven network solutions based on synthetic data.
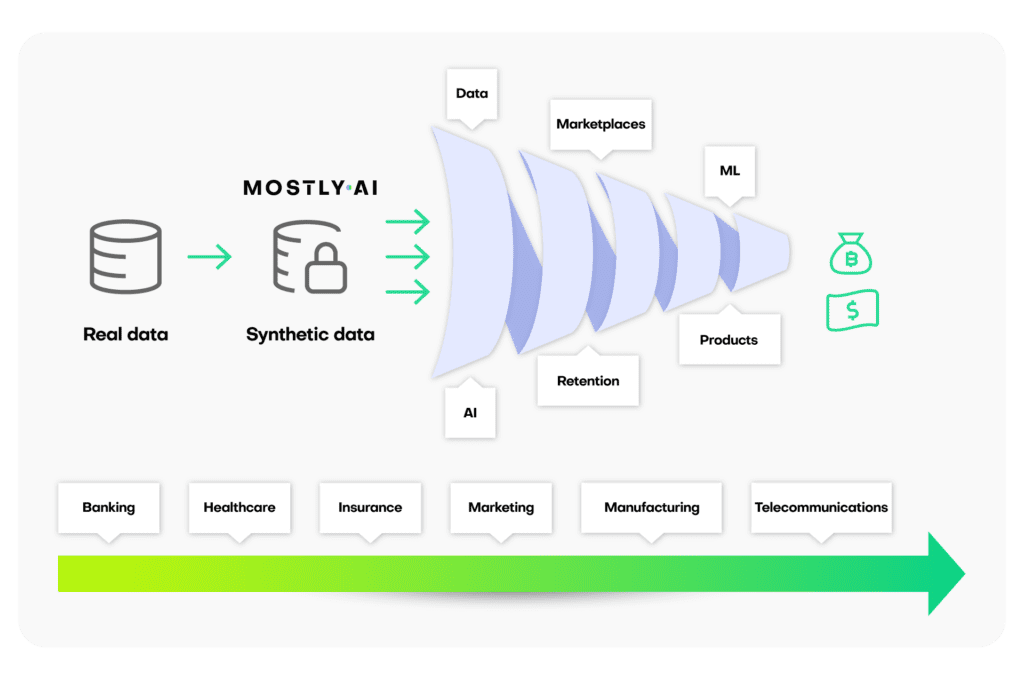
Conclusion
In this era of data-driven innovation, the power of synthetic data in data monetization cannot be overstated. It allows organizations to tap into the wealth of insights hidden within their data while upholding stringent privacy regulations and ensuring data security. From financial institutions fine-tuning credit risk models to marketing firms creating personalized campaigns and telecommunication companies optimizing networks, synthetic data is the key to unlocking new revenue streams.
For those seeking a private and secure synthetic data generation solution, MOSTLY AI's platform stands as a beacon. Our “private by default” synthetic data ensures that sensitive information remains protected while still enabling organizations to make the most of their data assets.
Ready to experience the benefits of synthetic data monetization with MOSTLY AI? Get started today by registering for our free version, and don't hesitate to contact us for more details. Your journey towards smarter, more profitable data-driven decisions begins here.