Last year MOSTLY AI has introduced and demonstrated the groundbreaking idea of generating fair synthetic data. I.e., data, that is representative of the real world, but that has unwanted biases, unwanted relations, surgically removed from it at the same time. Machine learning models that are then trained on fair synthetic data will be fair by design. It’s a thought-provoking paradigm shift, that will allow organizations to govern not only Privacy but also Fairness within AI at its source, that is the AI training data itself.
Fast forward to today, we are excited to see many things happening around fairness:
- The broad public interest in fairness and AI bias has drastically picked up, resulting in media coverage, documentaries, books, public debates, analyst reports, etc.
- The regulators are becoming active, with most notably the European Commission proposing an AI regulation, that explicitly demands that training data shall be fair & representative. US regulators are expected to follow suit, particularly within high-risk domains, like finance and health care.
- Leading AI conferences, like the ICLR, expand beyond accuracy and dedicate workshops to ethics, like Responsible AI or Synthetic Data for Privacy.
Speaking of ICLR, we had the honor to present our work on fair synthetic data at this year’s conference. This is another recognition of our work, which was already featured by Andrew Ng, IEEE Spectrum, Forbes, Slate, and many more. While the corresponding research paper is now available on arxiv.org, and the Fair Synthetic Data poster is accessible here, we summarize the key take aways once more:
- MOSTLY AI’s technology allows to generate Synthetic Data that is both statistical representative as well as fair - in the sense that it adheres to provided fairness constraints
- The trade-off between representativeness and fairness can be explicitly controlled for.
- Hidden proxy variables (e.g., body height serving as proxy for gender, or ZIP codes for race), which pose a significant challenge in combating biases, are successfully controlled for.
- And fairness can be established while only marginally impacting the downstream machine learning utility. One thus does not need to compromise significantly on accuracy in the pursuit of Ethical AI. Find out how to create fair synthetic data from our earlier blog post!
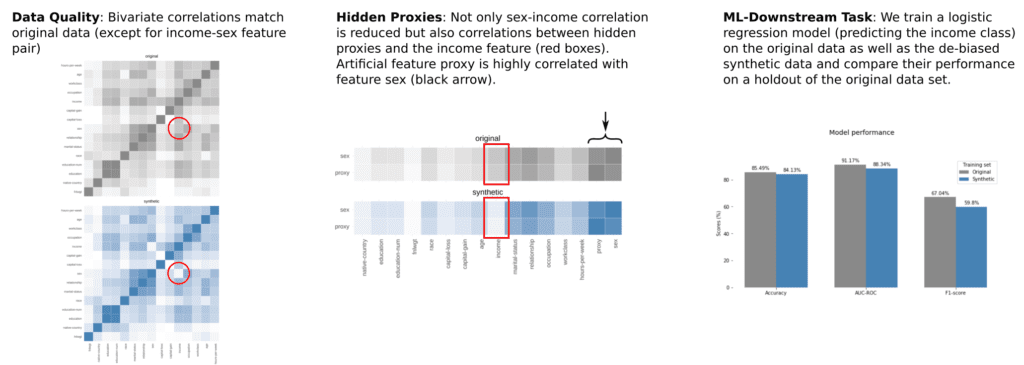
Last but not least, and as further testimony to the validity of the approach, Amazon just published a paper on fast fair synthetic data as well. And as their study leverages the same US census data as our paper, it allows for a direct comparison of results. We always knew that the quality of our synthetic data is unparalleled, but even we were amazed to see the effectiveness of our approach, as we excel on every single available dimension of realism, accuracy, as well as fairness:
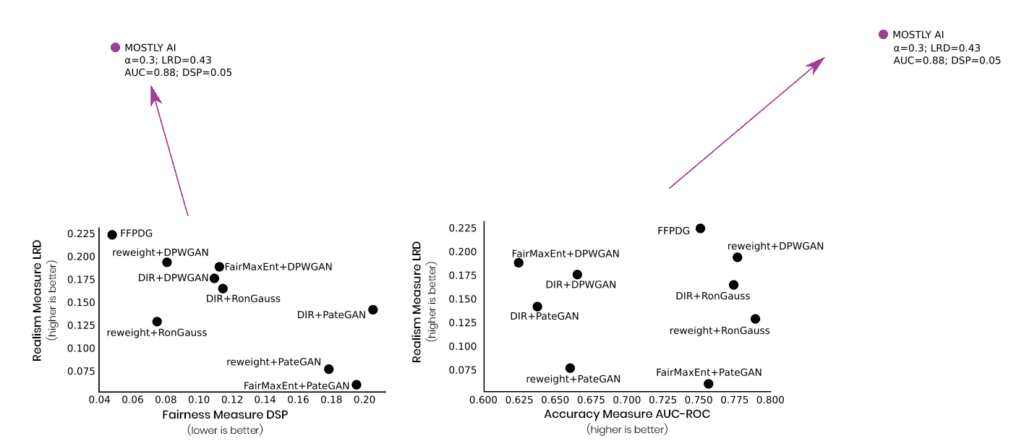
So, if Ethical AI is a priority for your organization or you deploy AI algorithms that directly impact the lives of individuals, then talk to us, and let’s discuss how we can get you started with fair synthetic data today. If you would like to learn more about fair synthetic data, read our Fairness Series!