Funding for digital healthcare has doubled since the pandemic. Yet, a large gap between digital leaders and life sciences remains. The reason? According to McKinsey's survey published in 2023, a lack of high-quality, integrated healthcare data platforms is the main challenge cited by medtech and pharma leaders as the reason behind the lagging digital performance. As much as 45 percent of these companies' tech investments go to applied artificial intelligence (AI), industrialized machine learning (ML), and cloud and edge computing - none of which can be realized without meaningful data access.
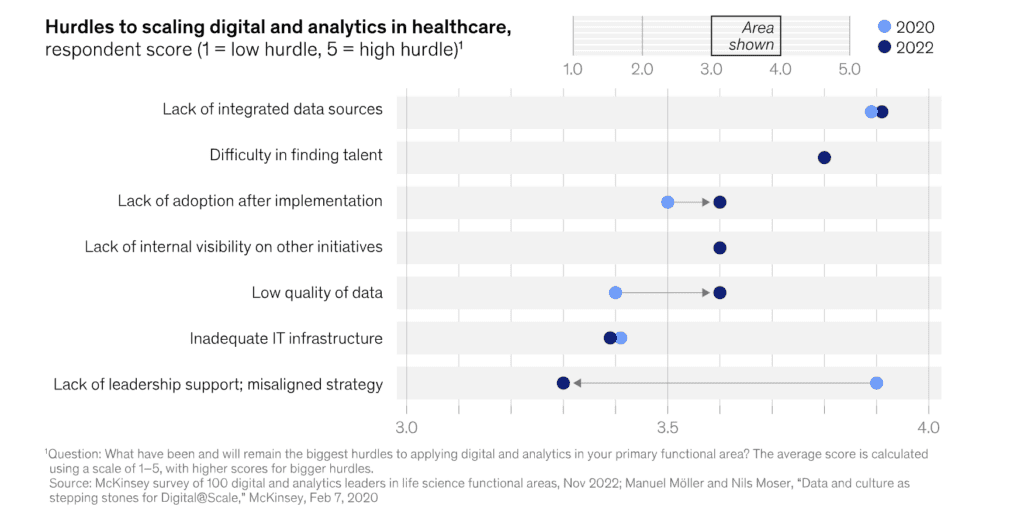
Why are healthcare data platforms mission-critical?
Healthcare data is hard to access
Since healthcare data is one of the most sensitive data types with special protections from HIPAA and GDPR, it's no surprise that getting access is incredibly hard. MOSTLY AI's team has been working with healthcare companies and institutions closely, providing them with the technology needed to create privacy safe healthcare data platforms. We know firsthand how challenging it is to conduct research and use data-hungry technologies already standard in other industries like banking and telecommunications.
Efforts to unlock health data for research and AI applications are already under way on a federal level in Germany. The German data landscape is an especially challenging one. The country is made up of 16 different federal states, each with its own laws and regulations as well as siloed health data repositories.
InGef, the Institute for Applied Health Research in Berlin and other renowned institutes such as Fraunhofer, Berliner Charité and the Federal Institute for Drugs and Medical Devices set out to solve this problem. This multi-year program is sponsored by the Federal Ministry of Health, a public tender MOSTLY AI won in 2022 to support the program with its synthetic data generation capabilities. The goal here is to develop a healthcare data platform to improve the secure use of health data for research purposes with as-good-as-real, shareable synthetic data versions of health records.
According to Andreas Ponikiewicz, VP of Global Sales at MOSTLY AI,
"Enabling top research requires high quality data that is often either locked away, siloed, or sparse. Healthcare data is considered as very sensitive, therefore the highest safety standards need to be fulfilled. With generative AI based synthetic healthcare data, that contains all the statistical patterns, but is completely artificial, the data can be made available without privacy risk. This makes the data shareable to achieve better collaboration, research outcomes, diagnoses and treatments, and overall service efficiencies in the healthcare sector, which ultimately benefits society overall”.
Protecting health data should be, without a doubt, a priority. According to recent reports, healthcare overtook finance as the most breached industry in 2022, with 22% of data breaches occurring within healthcare companies, up 38% year over year. The so-called deidentification of data required by HIPAA is the go-to solution for many, even though simply removing PII or PHI from datasets never guarantees privacy.
Old-school data anonymization techniques, like data masking, not only endanger privacy but also destroy data utility, which is a major issue for medical research. Finding better ways to anonymize data is crucial for securing data access across the healthcare industry. A new generation of privacy-enhancing technologies is already commercially available and ready to overcome data access limitations. The European Commission's Joint Research Center recommends AI-generated synthetic data for healthcare and policy-making, eliminating data access issues across borders and institutions.
Healthcare data is incomplete and imbalanced
Research and patient care suffer due to incomplete, inaccurate, and inconsistent data. Filling in the gaps is also an important step in making the data readable for humans. Human readability is especially mission-critical in health research and healthcare, where understanding and reasoning around data is part of the research process. Machine learning models and AI algorithms need to see the data in its entirety too. Any data points masked or removed could contain important intelligence that models can learn from. As a result, heavily masked or aggregated datasets won't be able to teach your models as well as complete datasets with maximum utility.
To overcome these limitations, more and more research teams turn to synthetic data as an alternative. Successfully implemented healthcare data platforms fully take advantage of the potential of synthetic data beyond privacy. Synthetic data generated from real seed data offers privacy as well as data augmentation opportunities perfect for improving the performance of machine learning algorithms. The European Commission's Joint Research Center investigated the synthetic patient data option for healthcare applications closely and found that (the):
"Resulting data not only can be shared freely, but also can help rebalance under-represented classes in research studies via oversampling, making it the perfect input into machine learning and AI models"
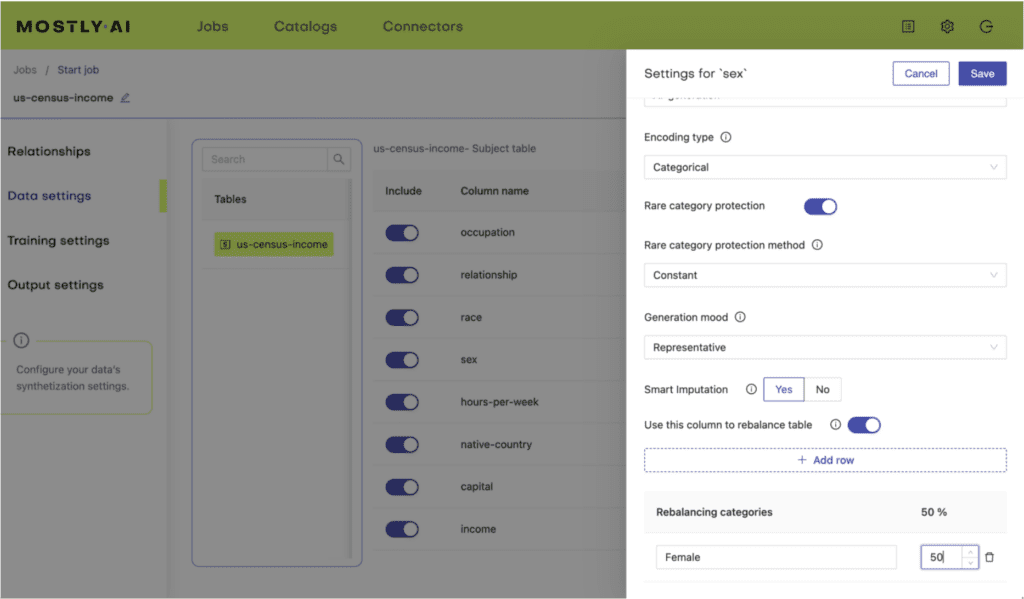
Healthcare data is biased
Data bias can take many shapes and forms from imbalances to missing or erroneous data on certain groups of people. Synthetic data generation is a promising tool that can help correct biases embedded in datasets during data preparation. The first important step is to find the bias in the first place. The more eyes you have on the data, the better the chances of identifying hidden biases. Clearly, this is impossible with sensitive healthcare datasets. Synthetic versions of data can increase the level of access and transparency of important data assets.
Health data is the most sensitive data type
A few years ago a brand new drug, developed for treating Spinal Muscular Atrophy, broke all previous records as the most expensive therapy in the world. If you were to query supposedly anonymous health insurance databases around that time, you could easily identify children who received this drug, simply because the price was such an outlier. But even in the absence of such an extreme value, reidentifying people by linking separate data points together is not a difficult thing to do.
Surely, locking health data up and securing the environment where the data is stored is the way to go. Except that most data leaks actually originate with a company's own employees. Usually, there is no malicious intent either. A zero-trust approach helps only to an extent and fails to protect from mistakes and accidents that are bound to happen. Locking data up also means fewer data collaboration, smaller sample sizes, less intelligence, higher costs, worse predictions, and ultimately, more suffering.
For example, the improvement of predictive accuracy of machine learning models regarding health outcomes can not only save costs for healthcare providers but also decrease suffering. The more granular the data, the better the capabilities in predicting how certain cohorts of patients will react to treatments and what the likelihood of a good outcome will be.
In one instance, MOSTLY AI's synthetic data generator was used for the synthetic rebalancing of patient records and predicting which patients would benefit from a therapy that can come with serious side effects. John Sullivan, MOSTLY AI's Head of Customer Experience, has seen how synthetic data generation can transform health predictions from up close.
"We worked on a dataset for a large North American healthcare company and achieved an increase of accuracy in true positives predicted by the down-stream ML model in the range of 7-13% against a target of 5-10%. The improved model performance means potentially hundreds of patients benefit from early identification, and more importantly, early treatment of their illness. It's huge and extremely motivating."
Considering that 85% of machine learning models don't even make it into production, synthetic training data is a massive win for data science teams and patients alike.
Synthetic healthcare data types
Different data types are present along the patient journey, requiring a wide range of tools to extract intelligence from these data sources at scale. Healthcare data platforms should cover the entire patient journey, providing data consumers with an environment ready for 'in-silico' experiments.
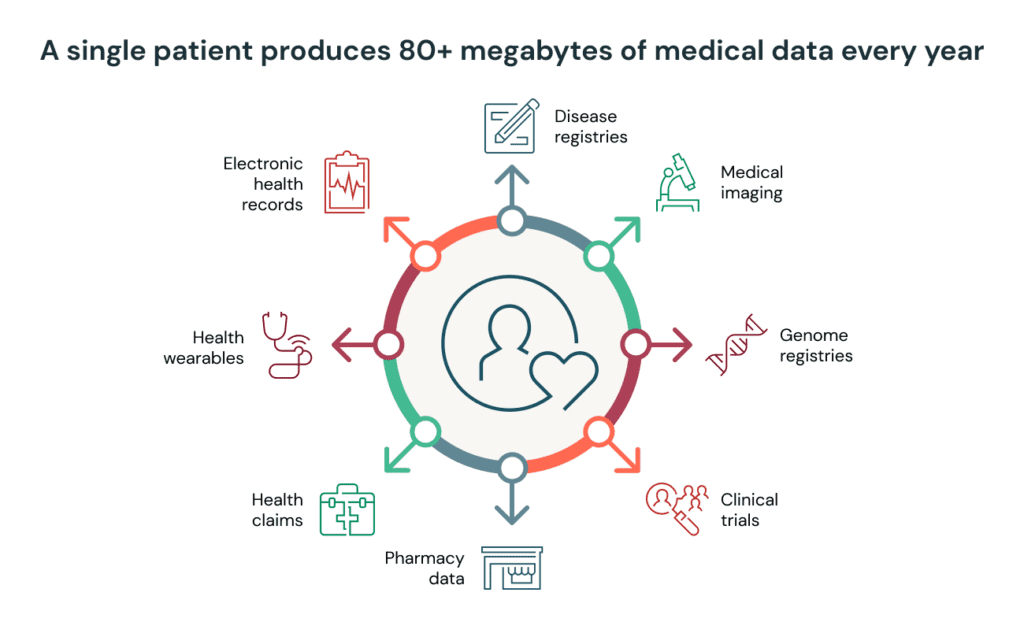
In healthcare, image data in particular receives a lot of attention. From improved breast cancer detection to AI-powered surgical guidance systems, medical science is undergoing a profound transformation. However, the AI revolution doesn't stop at image analyses and computer vision.
Tabular healthcare data is another frontier for new artificial intelligence applications. Synthetic data generated by AI trained on real datasets is one of the most versatile tools with plenty of use cases and a robust track record, allowing researchers to collaborate even across borders and institutions.
Examples of tabular healthcare data include electronic health records (EHR) of patients and populations, electronic medical records generated by patient journeys (EMR), lab results, and data from monitoring devices. These highly sensitive structured data types can all be synthesized for ethical, patient-protecting usage without utility loss. Synthetic health data can then be stored, shared, analyzed, and used for building AI and machine learning applications without additional consent, speeding up crucial steps in drug development and treatment optimization processes.
Rare disease research suffers from a lack of data the most. Almost always, the only way to produce significant results is to share datasets across national borders and between research teams. This process is sometimes near-impossible and excruciatingly slow at best. Researchers need to be working on the same data in order to make the same conclusions and validate findings. Synthetic versions of datasets can be shared and merged without compliance issues or privacy risks, allowing rare disease research to advance much quicker and at a significantly lower cost. On-boarding researchers and scientists to healthcare data platforms populated with synthetic data is easy to do, since the synthetic version of datasets does not legally qualify as personal data.
Use cases and benefits for AI-generated synthetic data platforms in healthcare
Let's summarize the most advanced healthcare data platform use cases and their benefits for AI-generated synthetic data. These are based on our experience and direct observations of the healthcare industry from up-close.
Machine learning model development with synthetic data
The reason why most machine learning projects fail is a lack of high-quality, large-quantity, realistic data. Synthetic data can be safely used in place of real patient data to train and validate machine learning models. Synthetic data generators can shorten time-to-market by unlocking valuable data assets by taking care of data prep steps such as data exploration at a granular level, data augmentation, and data imputation. Since data replaced code, healthcare data platforms became the most important part of MLOps infrastructures and machine learning product development.
Data synthesis for data privacy and compliance
Protected health information, or PHI, is heavily regulated both by HIPAA and GDPR. PHI can only be accessed by authorized individuals for specific purposes, which makes all secondary use cases and further research practically impossible. Organizations disregarding these rules face heavy fines and eroding patient trust. Synthetic data can be used to protect patient privacy by preserving sensitive information while still allowing for data analysis.
Testing and simulation with synthetic data generators
Synthetic data helps researchers to forecast the effects of greater sample size and longer follow-up duration on already existing data, thus informing the design of the research methodology. MOSTLY AI's synthetic data generator, in particular, is well suited to carry out on-the-fly explorations, allowing researchers to query what-if scenarios and reason around data effectively.
Data repurposing for research and development
Often, using data for secondary purposes is challenging or downright prohibited by regulators. Synthetic data can overcome these limitations and be used as a drop-in placement to support research and development efforts. Researchers can also use synthetic data to create a so-called 'synthetic control arm.' According to Meshari F. Alwashmi, a digital health scientist:
"Instead of recruiting patients to sign up for trials to not receive the treatment (being the control group), they can turn to an existing database of patient data. This approach has been effective for interpreting the treatment effects of an investigational product in trials lacking a control group. This approach is particularly relevant to digital health because technology evolves quickly and requires rapid iterative testing and development. Furthermore, data collected from pilot studies can be utilized to create synthetic datasets to forecast the impact of the intervention over time or with a larger sample of patients."
Population health analysis for policy-making
AI-generated synthetic data can be used to model and analyze population health, including disease outbreaks, demographics, and risk factors. According to the European Commission's Joint Research Center, synthetic data is "becoming the key enabler of AI in business and policy applications in Europe." Especially since the pandemic, the urgency to provide safe and accessible healthcare data platforms on the population level is on the increase and the idea of data democratization is no longer a far-away utopia, but a strong driver in policy-making.
Data collaborations for innovation
Creating healthcare data platforms by proactively synthesizing and publishing health data is a winning strategy we see at large organizations. Humana, one of the largest health insurance providers in North America, launched a synthetic data exchange platform to facilitate third-party product development. By joining the sandbox, developers can access synthetic, highly granular datasets and create products with laser-sharp personalizations, solving real-life problems. In a similar project, Merkur Insurance in Austria uses MOSTLY AI’s synthetic data platform to develop new services and personalized customer experiences. For example, to develop machine learning models using privacy-safe and GDPR-compliant training data.
Ethical and explainable AI
Synthetic data generation provides additional benefits to AI and machine learning development. Ethical AI is one area where synthetic data research is advancing rapidly. Fair models and predictions need fair data inputs and the process of data synthesis allows research teams to explore definitions of fairness and their effects on predictions with fast iterations. Furthermore, the introduction of the first AI regulations is only a matter of time. With regulations comes the need for explainability. Explainable AI needs synthetic data - a window into the souls and inner workings of algorithms, without trust can never be truly established.
If you would like to explore what a synthetic healthcare data platform can do for your company or research, contact us and we'll be happy to share our experience and know-how.