Prologue
In one of our earlier blog posts we demonstrated our synthetic data platform using a well studied, publicly available dataset of over 50’000 historic diamond sales with 10 recorded data points each. It served as an educational example to introduce the idea of synthetic data, as well as to showcase the unparalleled accuracy of our technology. With the click of a button, users of our platform can forge an unlimited number of precious, highly realistic, highly representative synthetic data diamonds.
But, to be fair, the example didn’t do justice to the type and scale of real-world behavioral data assets encountered in today’s industry, whether it’s financial, telecommunication, healthcare or other digital services. Organizations operate at a different order of magnitude, as they serve millions of customers and record thousands of data points over time for each one of those. Whether these recorded sequences of events represent transactions, visits, clicks or other actions, it is so important that these rich behavioral stories of customers are understood, analyzed, and leveraged at scale in order to provide smarter services with the best possible user experience for each customer.
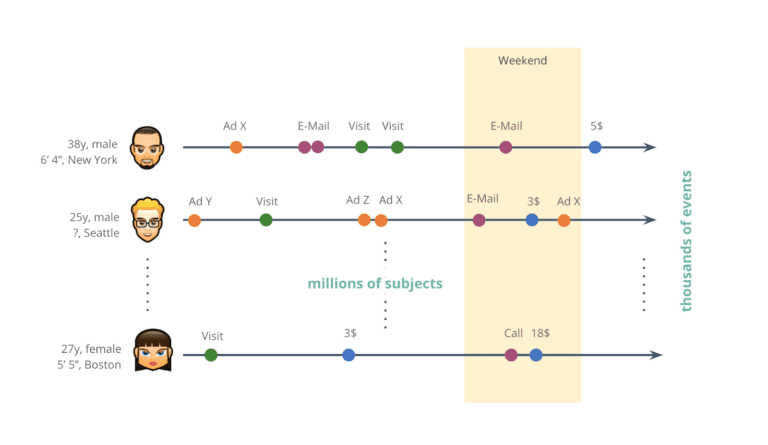
Today, organizations collect thousands of events for each and every one of their millions of customers.
But despite the immense growth in volume over recent years, the captured behavioral data still remains vastly an untapped opportunity. And over and over again, we can identify two key obstacles at organizations at play:
1) Behavioral data is primarily sequential and constantly evolving, rather than static and fixed – and with its thousands of data points per individual, there is a sheer unlimited number of potential temporal inter-dependencies and contextual correlations to look for. To say it simply: It’s a fundamentally different category beast than what is being taught at Statistics 101. Existing business intelligence tools, as well as regression or tree-based models struggle in making sense of this type of data at scale. Thus it is no surprise that only the most data-savvy organizations turn up on the winning side by knowing how to leverage their immense behavioral data assets to effectively gain a competitive edge with hyper-personalized customer experiences.
2) The second obstacle is, that behavioral data remains primarily locked up. Because with thousands of available data points per customer the re-identification of individual subjects becomes increasingly easy. Existing anonymization techniques (e.g. data masking), that have been developed to work for a handful of sensitive attributes per subject, stand no chance in protecting privacy while retaining the utility of this type of data at a granular level. A disillusion that is by now also broadly understood and recognized by the public:
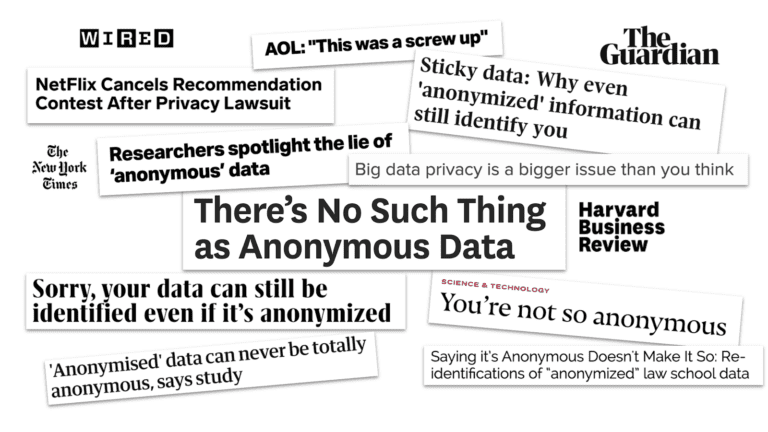
As it turns out, these are two reinforcing effects: Without safe data sharing, you can’t establish data literacy around behavioral data. Without data literacy, you will not see the growing demand for behavioral data in your organization. However, only some companies will remain stuck in their inertia, while others are able to identify and thus address the dilemma by turning towards synthetic data, which allows them to offer smart, adaptive, and data-driven services to win the hearts of the consumers (as well as the markets).
The Curse of Dimensionality
Let’s look at an example to illustrate the complexity of sequential behavioral data. Within retail banking, each account will have a sequence of transactions recorded. But even if we discard any personally identifiable information on the customer, and even if we limit the amount of information per transaction to 5 distinct transaction amounts, and 20 distinct transaction categories, the number of behavioral stories quickly explodes with the length of the sequences. While a single transaction seems innocuous with its 20*50 = 100 possible outcomes, two transactions will already yield 100*100 = 10’000 outcomes. For a sequence of three transactions we are at 100^3 = 1 million outcomes per customer, and at forty recorded transactions, we will already have more possible outcomes (10^80) than atoms in the universe! No wonder, that these digital traces are highly identifying, and near impossible to obfuscate. No wonder, that making sense of this vast sea of data and detecting patterns and nuances therein poses such a huge challenge.
This combinatorial explosion, the exponential growth of outcomes with the number of records per subject, is also referred to as the curse of dimensionality. There is no person like another, everyone is different, everyone is unique. It’s a curse for analytics, it’s a curse for protecting privacy. But, at the same time, it’s a blessing for customer-centric organizations, who are willed to embrace a rich, diverse world of individuals, and who recognize this to be an opportunity to differentiate on top of these otherwise hidden behavioral patterns.
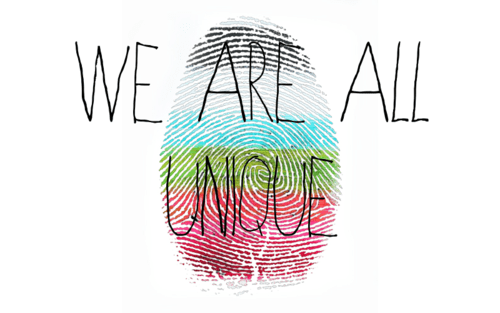
AI-Generated Synthetic Data to the Rescue
The power of synthetic data continues to be recognized as THE way forward for privacy-preserving data sharing. While there are various approaches and levels of sophistication, ranging from simple rule-based to more advanced model-based generators, our focus at MOSTLY AI has always been on offering the world’s most accurate solution based on deep neural network architectures. These are high-capacity, state-of-the-art machine learning models, that can reliably and automatically pick up and retain complex hidden patterns at scale. In particular for the type of sequential data, that is so prevalent among an organization’s behavioral data assets. These models make little a-prior assumptions and require no manual feature engineering by domain experts. They are the very same models that have revolutionized so many fields already over the past couple of years, like image classification, speech recognition, text translation, robotics, etc., that are now about to change privacy-preserving big data sharing once and for all.
And ultimately, it is the accuracy and representativeness of the synthetic data that is the key driver of its value. This is what will determine whether use cases go beyond mere testing & development, and expand towards advanced analytics and machine learning tasks as well, where synthetic data can be relied on in lieu of the actual privacy-sensitive customer data. And just as classic learning algorithms continue to be superseded by deep learning in the presence of big data, one can already observe a similar evolution for the market of synthetic data solutions for behavioral data assets.
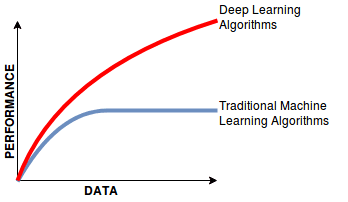
This was the first part of our mini-series on sequential data, setting the stage for next week’s post. There we will present a handful of empirical case studies to showcase the power of our synthetic data platform, in particular with respect to the important domain of behavioral data – so make sure that you don’t miss out on it!
Credits: This work is supported by the "ICT of the Future” funding programme of the Austrian Federal Ministry for Climate Action, Environment, Energy, Mobility, Innovation and Technology.