50. How to Democratize Data and Bridge AI's Tech/Business-Gap with Erste Group's Anna Lishchenko
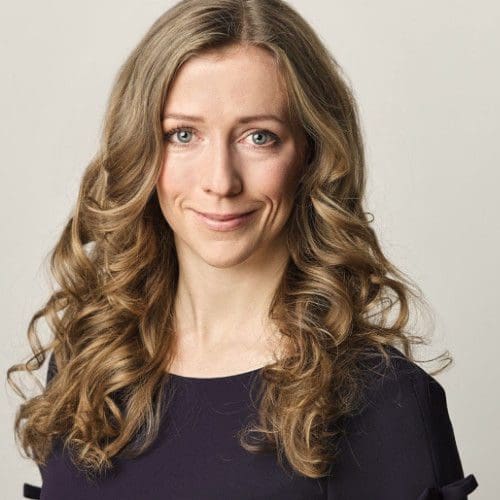
- The essential elements of building a data-driven culture, including data literacy, governance, and community building.
- Laying the foundation for success: How to align data and AI initiatives with business goals and secure buy-in from key stakeholders.
- Breaking barriers to experimentation: Using synthetic data to democratize access and accelerate innovation.
- Bridging silos: Connecting business and technical teams to drive collaboration and create real impact.
- Scaling AI and data initiatives: Turning one-off projects into sustainable enterprise-wide strategies.
Transcript
1
00:00:00,000 --> 00:01:25,839
Alexandra Ebert: Hello and welcome to the Data Democratization Podcast. This is episode three of our new season, but it's also the 50th episode that we ever recorded. So here's to that. Today I'm speaking with Ganna Lishchenko, who is the Data & Analytics for Business Platform Lead at the European bank Erste Group, and she's a true champion of data and AI democratization. So in today's episode, you will learn everything about data democratization from the foundations regarding establishing data literacy, building communities, and making sure that your data governance and data management supports your initiatives, towards navigating the challenges and intricacies when it comes to truly connecting AI and data innovation with the business line to make sure that it results in real impact for your organization. It's an episode packed with insights, and I'm sure there's a lot for you to take away from this episode. So let's dive right in. Welcome to the Data Democratization Podcast, Anna. It's a pleasure to have you on the show. I'm very much looking forward to our conversation today. But before we dive in, could you briefly introduce yourself and also share what makes you so passionate about the work that you do at Erste Group?
2
00:01:25,839 --> 00:02:43,039
Anna Lishchenko: First of all, thank you for having me, Alexandra. It's a pleasure being here. So a few words about myself. My name is Anna Lischenko, and currently I have a position of data analytics for business platform lead. But maybe the name doesn't tell exactly what I'm doing. So my main goal is to help organization to get the most value out of data. It's quite often in organization when we have data analytics projects, we quite a lot of time we're dedicating for technical part, but very often business part, not in the focus. But at the end, if business part won't happen, actually it won't succeed because we will not achieve business goals, what we put as an ambition. And it's important to put some emphasis on it. So this is I am exactly a person who tried to do it. It's not trivial task, I would say. And I think this is one of the reasons why it's not in the focus, because it's actually it's not so structured or not so clear what needs to be done. But what I see, it's a lot to do, actually, in order to make it happen.
3
00:02:43,039 --> 00:03:04,960
ALEXANDRA EBERT: I can totally imagine and I have plenty of more questions about your role being at this so important intersection between the business side of things and the data, AI and technical side of this endeavor. Before we dive into that, for those of our listeners who aren't familiar with Erste Group, could you give a brief overview of the organization, how it is set up, what it is about, just so that everybody can follow along?
4
00:03:04,960 --> 00:03:43,880
ANNA LISHCHENKO: Erste Group, it's a banking group, leading banking group in C region. So we're represented in seven markets directly and a few more in the Balkans indirectly. And we are talking about organization size with 46,000 employees. So our assets is 344 billion euros. And yeah, so it's not a small organization, we have quite some subsidiaries that we need to take care of. This is why it makes also complex.
5
00:03:43,880 --> 00:04:37,000
ALEXANDRA EBERT: I can imagine, particularly if you're set up in different regions, locations, then there are also some challenges when it comes to introducing AI and data driven initiatives to really decide what should be central, what should be decentralized. So we will definitely also get to that. But first to the other topic that I wanted to ask you more about, and hear it so often from people on my podcast, executives I'm working with, that it's this big challenge of once an organization wants to pursue AI and become more data driven, that they sometimes just have the innovation department or the tech department trying out these technologies in isolation, not necessarily talking to the business side of the organization, figuring out which use cases are actually moving the needle beneficial for the organization. So it's fascinating to see your role being at this exact intersection. Can you share how did this role come into existence?
6
00:04:37,959 --> 00:06:29,079
ANNA LISHCHENKO: I think our top managers realized that we are quite good actually on the technical side, and then we need another impact to happen that we see really that it's not we have just a very good stake of tools in place, but we really use it to achieve our business targets. Then it was the idea to create this role and to help organization to think what can be done. So also this role is pretty much connected with sharing of knowledge, because we have a lot of units, we are pretty much decentralized, I would say. From data point of view, we have very different business units, or what we call not exactly business units, but other units in the banks that are also working with the data. And so far they were doing quite well when they needed to deliver their own tasks and so on, but it was very little talk between themselves. And then if we will put on top also different countries, what is happening in countries to have a change of know-how between countries, it's becoming quite important to make it happen. To see overview what is happening, to connect people if they're working on similar problems, that they learn from each other, that they are not doing already mistakes or lessons, or they can benefit from lessons of other units. So this is quite important, I believe. Also can help to achieve certain targets faster, because you're simply not going the same journey all the time, but you can already learn from somebody.
7
00:06:29,559 --> 00:06:48,519
ALEXANDRA EBERT: I agree, I agree. So you mentioned earlier that this role is not super structured, but you would say then that your core responsibilities from what you just shared are connecting people, sharing the knowledge, democratizing AI and data, and also making sure that the tools are in place to make all of that happen. Or did I miss something super crucial?
8
00:06:49,959 --> 00:07:24,760
ANNA LISHCHENKO: Yeah, currently we are in the stage that we would like to boost a bit more use cases, what we're having. But in certain areas we're reaching that we have even too many use cases, and another part of the role will be to prioritize them. So to choose what is bringing more value to organizations. So it depends on where we are and what is our current challenge to help either to boosting more activity or other way around if we need to prioritize it. So we also focus where it matters.
9
00:07:25,480 --> 00:07:59,239
ALEXANDRA EBERT: Makes sense, makes sense. And I will definitely dive into use cases and try to pick your brain on that. But when we had our preparation call, you were also sharing, hey, you wouldn't position yourself and the organization as we've reached the end destination and we've achieved everything there is to achieve, which I think is where every organization currently is. It's a journey. But with that in mind, would you say that the role that you have is something that you believe would also benefit other banks, other enterprises to introduce, to really make sure that this important connect between business and data and AI technical folks happens?
10
00:08:00,440 --> 00:09:25,640
ANNA LISHCHENKO: Yeah, I believe so. I truly believe so, because very often on the business side, people have own agenda and sometimes they even not aware of possibilities what data brings. So there is someone who also understand business and their priorities, need to talk with them the same language and show and demonstrate possibilities that can be done. So actually, this person cannot do things by him or herself, but to help people to find ideas, to see potential, this is a possibility. And it will not happen by itself, unfortunately. So everybody's busy, they mind all business. And actually, even while demonstrating, also benefits not always coming smooth. You need to insist on certain things and it happens. So even if you see that people not reacting from first time because they're busy, they say, OK, maybe it's a good idea, but I have my other priorities. But if you insist and they realize, yes, it could help in my targets at the end. So why not to try it out? And sometimes people simply don't know. And when they don't know, they don't try it out.
11
00:09:26,599 --> 00:10:04,200
ALEXANDRA EBERT: Makes sense. Makes sense. I'm curious now, is the majority of your work more setting clear expectations and showing the possibilities of what can be done with data and AI? I know it very often from organizations that one of the challenges is that people either have overinflated expectations and are like, OK, we have one problem. Let's put AI to the test and let's use that or are super afraid and don't even want to go near these technologies. Or is it rather, OK, really connecting it to the business priorities of the certain business units to get them convinced that it makes sense to use data and AI to achieve their objectives?
12
00:10:07,000 --> 00:12:12,119
ANNA LISHCHENKO: I would say both. And here, if we're talking about AI, it's not only generative eyes. And recently, everybody's focused. But we're also part of AI with machine learning. And for sure, there are some people who still believe it's something magic can happen and can resolve all problems. So we need to continue actually literacy around the topic for people to make understand what is possibilities and what could be really done and what it's not possible actually to do now. And as you also said, it's quite important to link it to real problems of the business that they would like to resolve and to have a real case around. So it's not just something very abstract or it's just experiment for sake of experiment. Even I truly believe in experiments. And I think even if some of them might be unsuccessful, at the end, one out of 10 or one out of five will be very successful. And we really can make a difference afterwards. But I truly believe in use cases that are resolving real problem. And this also keeps people motivated to do things. And we are talking not about technical team who needs to develop it, but later to business really implement it. Because we also faced some situations that we agreed to do. For example, certain model was done and then business hesitating still, shall we implement or not? Especially, for example, we're talking about business talking to clients and we, for example, bringing models that indicates that certain clients will have propensity to buy certain product on something. But I know my client better. I don't believe for whatever model would give me some result. But to convince sometimes people even to try out results is also exercise by itself.
13
00:12:12,119 --> 00:12:36,919
ALEXANDRA EBERT: Yeah, I can imagine. And I will definitely also come to this aspect of you believing into experiments, how to actually do it right once we talk about use cases. But maybe first to give more context to our conversation. We know doing data and AI at scale, attaching it to business outcomes and achieving real value is a journey for every organization. What would you say are the most important elements for an organization to really get to that stage?
14
00:12:38,280 --> 00:13:48,440
ANNA LISHCHENKO: For sure, it depends where the organization are and at which stage and which challenges they're facing. But I would say what currently we see for ourselves, main areas where we need to invest on the business side in order that we can boost business value out of data. It's one thing, it's know-how exchange, what I mentioned, and I'm quite on it. Then I would say also still data literacy. And also, last but not least, data governance. Even some people, especially on business side, not always would like to hear it. But I also find it extremely important to have a very clear and transparent environment as different units can exchange data and data products amongst themselves. Without this framework, it simply won't happen.
15
00:13:49,640 --> 00:14:09,320
ALEXANDRA EBERT: Makes sense, makes sense. So it's basically this aspect of democratizing access to data, making sure that the tools are available, the data is available, and not only a few expert people, but basically the majority of the organization is really empowered to access these tools, access the data, access AI and build their own solutions with it.
16
00:14:09,640 --> 00:14:46,919
ANNA LISHCHENKO: Exactly, especially if the organization is fairly decentralized. So when our organization is more centralized, usually for them things are happening easier. But they, I think, are facing other kinds of challenges. They could be too far from the business at the end. And I believe that it's quite good to have people who are doing data products closer where they will be then further implemented and used. So in this respect, connecting or creating that network, it's quite important.
17
00:14:49,000 --> 00:15:11,080
ALEXANDRA EBERT: Definitely. And maybe also to clarify this for our listeners, on the one hand, I just recently was at Money 2020 in the United States, where every executive on stage talked about this importance of democratizing data, democratizing AI. Now you mentioned data products. So in your mind, what's the difference between giving access to data versus really giving access to data products? And what are the different benefits from that?
18
00:15:13,000 --> 00:16:20,440
ANNA LISHCHENKO: The biggest difference from my point of view is that data product has a data owner who takes care that data product is a good shape. And also managing stakeholders, meaning not just you get access to certain data and you don't know what is happening, if it will be good or not. Somebody who takes care that it's in good shape. If any changes will happen, this person will communicate to all stakeholders that they can take it into consideration. Also, that it's up to date, if anything changed, that it's still working. Also, if something happens with a product, somebody is there to fix it. So it's about, I think, creating trust in what people are using. And this can help to not to build everything from scratch, but create certain building blocks that people can ready to use and start to build their own data product. And it's simply making happening faster.
19
00:16:21,479 --> 00:16:36,039
ALEXANDRA EBERT: Yeah, I can imagine that if you have this trust that there's proper data governance, data management, the data will be always up to date. That this really helps you to then take what's already been done, particularly with this decentralized organization and incorporate this according to your needs.
20
00:16:36,599 --> 00:16:47,479
ANNA LISHCHENKO: Yeah. And especially if you're talking also cross countries, certain things also can happen. It's not only within one organization between different units, but we're also talking across
21
00:16:47,479 --> 00:17:11,319
ALEXANDRA EBERT: borders as well. Makes sense. Makes sense. You also mentioned this important concept of data literacy. And I'm curious now because some argue, well, now that we have Gen AI tools and everybody can have their coding or data assistant next to the site, do we even need to have data literacy? And how, in your opinion, should data literacy look like for different roles that you have within such a large organization?
22
00:17:12,280 --> 00:18:48,439
ANNA LISHCHENKO: Yeah. Some people are even allergic to this word of data literacy because... Really? Okay, yes, I understand why you tried to teach me. But at the end, if you start to talk to people and say, okay, things may be a little bit different or explaining and they understand that topic is much deeper than they thought. And it's quite important, I think, to invest in this education, first of all, of wider even population of organization, not only people who are dealing with the data, meaning data scientists, data analysts, data engineers, but we're also talking about people on the business side that they understand how models, for example, working or how AI is working, what is possible, what is not possible, what is, for example, downsides of the things that we can manage risks properly and so on, to manage also expectations, what can be done, what is not. And for them, understanding the thing, they also can come with good quality ideas from business side what can be done. So I think it's beneficial from both sides, from data people to understand business very well and they also can create a better quality of the product. But from another hand, it's very important people on the business understand how data works. It is not a black box for them. That is something they know how it's functioning. And then I think, first of all, will be more ideas, better ideas, and I think it will be simply faster delivery.
23
00:18:49,880 --> 00:19:04,780
ALEXANDRA EBERT: I totally see that. Can you share how did you implement data literacy within the organization? Was this a central approach? How much executive support was there? How many incentives were necessary for people to even engage with this topic and learn about it?
24
00:19:06,280 --> 00:21:39,160
ANNA LISHCHENKO: Currently, we have a very different kind of trainings that people can do either online or in class. I would say if you look on the countries, it's still done quite decentralized. So each country also do it in their own way. But certain things that we did in headquarters are also available for people in countries. We just still need to boost also to make people aware more what is possible and how they can do. And for sure, we have a very different kind of specialized training, especially for people on the data side. So in cooperation with vendors or in cooperation with third-party providers who specialized really on training people getting certificates and things like this. And also what we use quite heavily now, it's communication channel because it's not very structured like training, but still also even to keep people aware of what is going on or also when we can share our successes or sometimes even lessons learned. We use quite heavily our communication channels like we have internal social network where we have, first of all, a community of data people, but also we can post for whole population regarding data and they can learn out of it. Community, I find quite important not only for data literacy, but also for know-how exchange, especially this channel with people passionate about data. We have now around 700 people in community. So it's quite a community, I would say. And I'm happy to see that it's not only admins who bring in content, but people already pick up the idea and they come with content themselves. They happy to share if they, for example, saw interesting article or is this interesting event or also they would like to share what they managed to achieve. And then I see that other people connecting to them. So it's not need to be done just through a bottleneck here in headquarters, but people start to communicate between themselves and it's the most important thing.
25
00:21:39,160 --> 00:22:16,359
ALEXANDRA EBERT: That's fascinating to hear, particularly given this decentralized setup. And when we had our preparation call, you also mentioned it's this challenge to make sure that data people, if they're sitting throughout the different business teams, don't feel isolated and really have this community feeling. So congrats on building up this lively community. I think many of our listeners at this point in time are wondering, how did you achieve that? How did you get people that sometimes might not be that open to share their successes, share, talk about what they're engaging with to really integrate this into their work life and sharing these successes via the social network?
26
00:22:18,439 --> 00:23:32,520
ANNA LISHCHENKO: True. Data people, it's very often introvert and it always would like to share. So it's about also creating for them environments that they feel like they're in a family and they can open up. But generally what I would recommend also to other colleagues to team up with their communication colleagues if they have such in organizations that they can recommend them which channels could be used. So currently we're actually leveraging all channels possible. It's not only the social network. For example, we have once per year big conference where people can meet in person and exchange know-how. So we have a lot of sessions with people. We really do demos and show what was done. Then also we organized a talk on know-how sharing when we see that some countries or some unions are working on the same topic. Then for sure social network, what I mentioned, we're also thinking about one common space where we can store a lot of information that people can come at any moment and read simply to see
27
00:23:32,520 --> 00:24:10,680
ALEXANDRA EBERT: what is happening. So basically an internal wiki or something like that? Yes, yes, yes. Things like this. Okay. And the conference you mentioned, is this again then exclusively for data professionals or do also business people come to that conference? Because I'm curious, you mentioned it earlier, you also use it to communicate to the wider organization online or internally with your social network to make sure that data literacy initiatives are not a one-off but the organization actually continues to transform. So is this conference something where business folks and data professionals connect or do you have other initiatives to really make sure that this continuous exchange happens?
28
00:24:11,319 --> 00:24:51,720
ANNA LISHCHENKO: So currently it was more focused on data professionals, to be honest. Even we had some business people also who are quite passionate and curious. But we are thinking for next year actually to change this proportion and also to have much more business people for the event. And also maybe to adapt a little bit formats that will help exactly this exchange. And for them getting ideas and also owning these ideas later and so not just like, okay, I think, but really, like, we have consequences out of it, I mean, in a positive way.
29
00:24:51,720 --> 00:25:05,160
ALEXANDRA EBERT: Fascinating. And are these conferences, just thinking back to a conversation I recently had, are these conferences also where you bring in external people, external experts for your team to learn from? Or is it more internal success stories and lessons learned?
30
00:25:05,160 --> 00:25:47,440
ANNA LISHCHENKO: So we also invite usually 4K and old speeches, either some vendors or so, or some people who are VC, could be interesting, could share something from the stage. For sure, we also have top management on the stage for people also to get motivation and also to understand where are we heading to, so what are our strategies. So yeah, it's combination. And I think it's a very good idea to combine both to have something from externals, something to learn about. And also to have a possibility to have know-how exchange.
31
00:25:47,440 --> 00:26:20,099
ALEXANDRA EBERT: Absolutely. And the reason I was asking you this, because when I was at Money2020 in Vegas at this large banking conference, I had the possibility to talk with somebody from Databricks. And once we talked, he was like, hey, I was just in Vienna recently. And then I was surprised to learn that he was actually a part of the conference. And he was so stunned because in his role within Databricks, he sees banks from around the world. And he says, rarely ever has he encountered an organization that is so aligned behind the vision towards data democratization, bringing AI to the organization, as that's the case with Erste Group.
32
00:26:20,099 --> 00:26:29,860
ANNA LISHCHENKO: So I was super positive about this conversation. Session from Databricks was very good. Yeah, yeah. People really enjoyed it.
33
00:26:29,860 --> 00:27:16,100
ALEXANDRA EBERT: I can imagine. But also his feedback, I think, is a testament to what Erste Group has been doing in the past and where you're already on your journey. So congrats to that also. Thank you. And now that we've talked about these, I would say prerequisites for doing data and AI at scale, making sure that this is not a one-off initiative, dies in the innovation centers or people get educated, but then never implemented to the business side. Data literacy, data governance, data management, building communities, having this continuous know-how exchange. Let's talk a little bit about the making data available puzzle piece of data democratization. Why is it important, in your opinion, to make sure that data is a resource that not only a privileged few people can access, but that's actually distributed throughout the organization?
34
00:27:16,460 --> 00:29:22,100
ANNA LISHCHENKO: First of all, in order to make things fast, because we are a bank, and as a bank, we quite care about our reputation. This is why for us all processes around accessing data, it's quite strict, I would say. And this is why it's taken for people sometimes quite a long time to get to the data. It's not technical. I think it's more organizational reasons. And here, actually, we can talk about MOSTLY AI, because we also have initiative currently to create some synthetic data sets where people can much easier to get access much easier and can do experiments right away and not spend quite some time for getting access first. Because people might think twice if you need to go through the process of getting access and say, OK, maybe should I try it or not? And we would like to boost these experiments more and to make it much easier for the people. And I think it will be a good source for good ideas, for new ideas. Because something what is already on the surface, I think, more or less was done. I would say we are quite good in this. And what we think about, like standard data scientists models for banking, I think we are all there. And we are thinking like to find something that is new, where we can be unique. And for this, we need for sure experimenting. You cannot do it otherwise. Just come up with it. And I see one of the keys to it as one of the solutions, have possibility for people to have quick access to data and synthetic data could be one of good solutions, especially when we try to really protect the data for our customers.
35
00:29:22,100 --> 00:31:03,980
ALEXANDRA EBERT: And on the same time, make it available. I also think back to a conversation a while ago I had with another organization we work with there in the telecommunications space. And they also built this internal synthetic data hub to make certain data sets widely available and make them accessible to also experiment and validate ideas with. And the person I was talking with was also, hey, I can't even remember the times before having synthetic data, because back then it was having an idea, requesting access to data, a lot of back and forth and maybe three months, six months, depending on the sensitivity of the data set. He finally got access to the data, versus now with this internal synthetic data hub, he can just access certain data sets automatically and validate his ideas. And coming back to this point of particularly when doing AI, but of course, also with other data initiatives, having the ability to experiment, I think synthetic data is also quite interesting tool because in contrast to legacy anonymization, where you have to say, okay, from all these hundreds of columns that I have, please give me column 5, 17, 21, etc. With synthetic data, you can make all the columns available. And then you can also really go into experimenting and figuring out what are actually the data points that I need, particularly for machine learning purposes, to come to a certain result and to achieve a certain outcome. So really curious to see what this initiative will bring within Oerster Group and how it helps you towards this journey. Other than that, where would you say is Oerster Group currently? You mentioned you've done many of the standard models in banking. So where would you say is Oerster Group currently on the journey for data democratization, AI democratization, and making sure that those technologies are used at scale within the group?
36
00:31:03,980 --> 00:32:31,700
ANNA LISHCHENKO: So currently, we're also working on implementing data product approach. So what we talked about changing governance, so we had very good practices for governance for regulatory part, but we would like to now to enhance it also to the business part. For sure, we don't want to do it one-to-one, because for regulatory, it's quite heavy processes that helps to make data perfect from quality point of view, from data lineage point of view. On business side, we don't need it to such extent to be so perfect. So we can for sure have a high degree of flexibility. So we are thinking now how to extend data governance and how to find this balance, from one hand, to be to create a safer space for people to work with data from different areas and they can rely on it. But from another hand, not to overdoing it, meaning that we don't invest too much in keeping it in good shape. So we are now currently in the process of finding this balance and for sure also leverage this idea of data products that we need to have ownership, that we need to have all metadata around data products and all processes around it. It's currently not as much structured as we wanted. So it's more case-by-case basis and we would like really to create as a framework for this one.
37
00:32:31,700 --> 00:33:03,940
ALEXANDRA EBERT: Fascinating. And maybe now coming to the use case part of our conversation, you already shared it earlier how important it is to attach a use case. What's your recommendation to our listeners, how they can really identify high impact use cases? And maybe, because I would assume you would say it depends on the stage of the organization, how could organizations that are maybe just starting out, let's say with AI, identify good use cases? What's your experience on that versus how do you currently on this much further maturity level approach identifying new use cases with the NERSD group?
38
00:33:03,940 --> 00:35:35,719
ANNA LISHCHENKO: When you have too many, especially, and you need to choose. So the best idea to start to look at what is strategic targets of organization and to have a link and see which initiatives help you to achieve strategic targets. Because for sure, experiments can be in various areas. It might not be directly linked, but with those who reach a link to strategy, for sure you will see impact and also for organization feels that we are moving in the right direction. So it's not just innovation for sake of innovation, but it helped us. Second thing maybe to look at for sure on the impact that it brings. So it's not just if it's connected, if it's really make a significant difference for organization as a for additional business or to achieving certain efficiencies. So to look on the size of could be financial or not financial benefits, what you can get. And also I think quite important to have a look on possibility to reuse after what is buildings that is not resolving just particular problem, but to build a solution in the ways it can be used for other use cases. Because it will be as you get value not only once, but you building possibility to use it more and bring even more value further. So I would focus on those initiatives that are really making impact. From another hand, it's also important to see how ready you are for them. And in case if you have all data in place, if your infrastructure it's or if also from architectural point of view, it makes sense that it's not ruin all the architecture. And also if you have all necessary skills in place, because if it's super good idea, you see that this will be very aligned with your strategy and also might have a significant impact. So if you have issues, for example, that you don't have skills or you don't have data, think about first to resolve this prerequisites and then to start. Because otherwise it will be too long. People might get upset that we are not progressing. Also it would be very difficult to manage expectation of stakeholders. So it's important maybe to do this homework to be prepared to start.
39
00:35:35,719 --> 00:36:07,320
ALEXANDRA EBERT: Yeah, I totally see that. And I think it also turns into what you earlier said about making sure ideally having a scenario that you then can repurpose or slightly change to get additional value out of it. Also, one of the keynotes at the Money2020 conference, the chief AI and data officer of Mastercard was very big on this point to really scale data and AI, how important it is to have this reusable and repurposable components to make sure that you're not always have to start from scratch and have to invest the resources there. So really fascinating to see how you approach that.
40
00:36:07,320 --> 00:36:51,479
ANNA LISHCHENKO: It's really a change of mindset. Even if you have all the tools in place and even if you have, for example, you created already all the rules in place, but it will not happen automatically. So you need to preach, you need to explain, you need to insist and it will happen. But only it's sometimes about patience and about time. Explaining people that, yeah, things should work differently. And please think not only about your problem now, but please think a little bit beyond it and think about organization as a whole, how it can benefit out of what you're creating, not only once, but more time.
41
00:36:51,479 --> 00:37:15,799
ALEXANDRA EBERT: Of course, this transformation is definitely a journey. Out of curiosity, is this preaching that you have to do and many of your colleagues, something that's more targeted towards potential users, business users of the data products, the AI products, or is it also continuously necessary to convince executives to stick to data and AI initiatives, even though you don't maybe see results in the first week of starting a new project?
42
00:37:15,799 --> 00:39:02,359
ANNA LISHCHENKO: I think both, actually. So it should be happening on many levels. So for sure, executives very often are impatient and say, OK, here is cool stuff. When they will see results, they are very impatient and would like to see it this up. And sometimes things simply take longer. As we said, for example, even data access is taking quite some time. Sometimes we really need to try out things. They might not work from the first try and we need to do several iterations. And it's fine because also quite now this field is so dynamic and so many things are coming. So it's fine to learn and make mistakes, but it's important to progress. So for sure, managing expectations of executives and keep them informed, quite important. And I believe it's also quite important to inform them not only about successes, but also about lessons learned, because it's also an investment in the knowledge in organization. So not only success in bringing knowledge, but also lessons learned, also bringing a lot of knowledge, even if you don't see it materializing in some particular value right away. But it makes you faster and to the point for any other initiatives that you will do further. And I think it's quite important to communicate to executives, also for important stakeholders on the business side as well, to say, OK, maybe we failed now, but we know we shouldn't do things like this anymore. So we do it differently now. And here is our success. So it should be a combination of both successes and lessons learned.
43
00:39:02,840 --> 00:39:15,440
ALEXANDRA EBERT: I think that's a very, very valuable recommendation. Other than that, any other recommendations you have from your experience that you want to share with our listeners when it comes to first getting buy-in from executives and then continuing to hold that buy-in?
44
00:39:16,440 --> 00:40:14,320
ANNA LISHCHENKO: For sure, it depends on the stage of organization. At least I was not in situations that I need to get buy-in of executives just from scratch. It's already somehow in their minds that data and analytics, it's very important and we should do it. Maybe they quite often don't know how it should happen. And this should be a role of people like me or also, for sure, colleagues on the IT side that we combine forces and brings them a really clear picture of where we would like to heading, what is our ambition, what needs to be done for this, what is our ambition business-wise, what is our ambition technically-wise and things like this. So we are here to help executives to make this thing clear and understandable what exactly can be done and what we aim to.
45
00:40:14,960 --> 00:40:18,799
ALEXANDRA EBERT: Makes sense. And then also how long it will take, the nature of experiments.
46
00:40:18,799 --> 00:40:54,880
ANNA LISHCHENKO: And I would say to continue because things, as I said, it's so dynamic and things are changing so fast. So I think we also should be in charge to keep them up to date, what is happening and if we also need to even review target pictures that was created, maybe even like one year ago or maybe like six months ago, to keep it up to date. And it's fine to change it because it's quite dynamic now and it's not a problem. I don't see a problem to change target picture if we have new technology in the place or things are simply changing.
47
00:40:55,760 --> 00:41:43,559
ALEXANDRA EBERT: Yeah, I would say that field we're in is really one where lifelong learning is of the essence and then also implementing that. I said earlier, I really wanted to pick your brain on the nature of setting up successful experiments and thinking to this number that's oftentimes circulated from analyst firms of, OK, 70 percent of AI projects are failing and oftentimes this connects to particularly this disconnect between just experimenting, playing around with the tech and not connecting it to the business side. So from your experience, what are really the steps our listeners should go through when starting with experiments to make sure that they set themselves up for a successful experiment, either because it's everything works as intended or because it's valuable to get some good learnings out of it and avoid just playing around with the technology and then not really getting business value out of it?
48
00:41:44,479 --> 00:43:36,079
ANNA LISHCHENKO: I think it's important to start experiment if you would like to see it materialize afterwards. It's to get the business buy-in from the beginning. So you need somebody on the business side that said, yes, you will develop and I will try out in business. And it's not always easy, to be honest. And even sometimes you seems like a good buy-in, but it's not always a case. So I think it's one thing that just it's not develop something and then it will lie on the shelf. So you need somebody who already said, yes, yes, I will try it out. And I think it's fine that not all experiments are successful as well. They also need to be open if something will not go right. Yeah. This is why it's experiment. It's just I think first of all, I think when we set up an experiment, for sure we would like to aim at success. But from another hand, we need to keep us open minded to whatever happened and to, I think, value learning more than maybe final outcome. I'm not sure if it really helps to achieve success, to be honest. But I think in the long run, it is so not for maybe for this particular experiment. But if you're talking about experimenting area, I think it will be much more beneficial. But currently, I see that I think the biggest problem of why certain experiments are not going live, it's because it's done in disconnection with business.
49
00:43:36,560 --> 00:44:10,000
ALEXANDRA EBERT: Yeah, yeah. And I think it also depends on this definition of definition of what is a successful experiment. Of course, everybody would hope that what they set out to do and achieving result X, Y, Z is then what is the result of the experiment. But also having an experiment that fails, but having valuable learnings that either help you to correct course or do better in the long run, as you just mentioned, I think is also something that can be classified as that's a successful experiment because at least you got valuable learning out of it and not just waste the time on something that the organization actually doesn't need because there is this disconnect.
50
00:44:10,800 --> 00:45:06,199
ANNA LISHCHENKO: I fully agree with you for learning, but also regarding if you talk about business, for example, it's also what I saw was very helpful to have a kind of already business ambition, even if it's experiment, if it's not final project, but still think what is hypothesis we would like to prove, what it will change for us business wise. And it helps you still to focus really on achieving something when you have from every beginning, not just, OK, maybe it can help me to automate something, for example, if you think about GNI, but to have a little bit more as articulated to the team as a business target, help them also to structure their minds to resolve this problem and not just trying out new technology or things like this. I think it's also quite important. And I not always see that people do so.
51
00:45:06,800 --> 00:45:27,199
ALEXANDRA EBERT: It makes sense. I always wanted to come back to this point of getting buy-in, not really having buy-in from your experience. If you really want to make sure that there is buy-in and that the experiment will be then at least tested from the business side, what are the steps that our listeners should go through? What are the checkboxes that they should try to tick to make sure that they really have good buy-in?
52
00:45:28,520 --> 00:47:43,119
ANNA LISHCHENKO: I think it's very dependent on the person with whom you talk. So you need to know a little bit better your counterpart with whom you are talking. I don't think it's one exact algorithm that you need to follow for this. There are people who are more open-minded and for them much easier to jump on the topic. And, you know, also based on experience, if a person says yes, then it really means yes. And also there are different colleagues who, if you see that people are hesitating, it's I think quite important to clean out from every beginning this hesitation and their concerns and try to understand what bothers them to do it before you start. This is helpful because if you have a very clear yes and you can trust on this yes of this person, then OK, you're done. But in case you see that there is a hesitation, I think it makes sense to invest time for clean up this concerns of the person and it will be much easier to go directly to implementation and not to start this hesitation when product was developed, because it could be that even these concerns could be very valuable concerns and it could be your input for your even data product development. So I think this communication is also quite important and also building a trust. And I think also during development, it's important to keep also contact with a business person to up-to-date where you are, how you're progressing, and also a possibility to clean up if anything appears also on the way, new concerns or certain topics so that you can clean it during the process and not after when it's much more difficult. And also help people from business to own data product more or also own this use case, not data product, but use case to own. And involvement helps a lot, I believe.
53
00:47:43,160 --> 00:48:15,079
ALEXANDRA EBERT: Yeah, yeah, I can totally see that. So I think once again, this emphasizes how important good communications and people's skills are to really make sure that data and AI are something that are used within the organization and can be used at scale. I think we could continue this conversation for quite a long time. There's so much that our listeners can learn from you. But since we have to come to an end, maybe two final questions. First, if you look ahead, what are the things that excite you most about the future of data democratization and using AI at scale within Earth to Group or beyond?
54
00:48:18,560 --> 00:48:19,599
ANNA LISHCHENKO: Not easy question.
55
00:48:21,079 --> 00:48:26,079
ALEXANDRA EBERT: Maybe you have some crystal ball lying around that can tell you more about the future.
56
00:48:28,560 --> 00:49:26,680
ANNA LISHCHENKO: I think we just need to continue what we started. We started a lot of things and my role is relatively new. So I started just the beginning of the year and it's still a lot to do. And we see a lot of tasks ahead of us. And, you know, you go, you reach a certain point and you see even a bigger area to do. So for me to say exactly what we will do in the future is very difficult. Currently, I think it's to implement a plan what we put for ourselves. And I already mentioned main areas what we are focusing. It's about know-how exchange, about data literacy, about setting new governance. And then we will see afterwards. So then when you solve one problem, you might see that other problems were not visible so much. And we need to tackle them.
57
00:49:27,680 --> 00:49:32,000
ALEXANDRA EBERT: So the conclusion is I need to invite you back again in one year or two years.
58
00:49:32,000 --> 00:49:50,560
ANNA LISHCHENKO: Or maybe I hope that we will have, because as I said, we have in certain areas quite a lot of use cases. But in certain areas, I would like to see much more of them and I would be really happy if even in the future we will have a problem of too many use cases that we really need to prioritize. This would be my wish. But now you
59
00:49:50,560 --> 00:49:55,840
ALEXANDRA EBERT: got me curious, what are these areas where you would love to see more use cases? It's on classical
60
00:49:55,840 --> 00:51:06,559
ANNA LISHCHENKO: machine learning and currently we did a lot of basic stuff but I think we didn't uncover fully potential there still and I think we can do much more and what I also like when I see that one or another countries have idea and they did it and it's quite unique so not so many other units were thinking about and I think here it's quite key knowledge sharing. And also what I would like to also to do is that we do not knowledge sharing just from data scientists to data scientists that only data people exchange knowledge. What I would like to leverage more that we actually do exchange of know-how from business who use data and have successful use case that they talk to the business counterpart. I think it will be much more powerful because they also see already results of implementing this and they also speak the same language because it's I think actually much easier to get I think data people to talk to each other they generally usually curious and would like to learn and so on for them it's not a problem but to bring business together to talk
61
00:51:07,120 --> 00:51:39,440
ALEXANDRA EBERT: it's another challenge on the way. I totally get that but I think there's so much value behind that that those who really see the results and are kind of converted to make sense to utilize these technologies data products etc to share with others what the successes were that they achieved they're wonderful. So final question for you Anna there's so much knowledge that you shared with us already today but if you have one last piece of advice for people who work in similar roles like you trying to really gap this common disconnect between the business side and the data and AI
62
00:51:39,440 --> 00:53:02,080
ANNA LISHCHENKO: side of things what would that advice be? So it's important communication so try to use any opportunity to communicate could be structured unstructured and also for example formal or less formal so just talk so human interaction helps a lot and just also not forget to invest time in this and very often we are busy with a project and we are forgetting to communicate to communicate sometimes our successes or also lessons learned to really to get a contact with the people on business side because it's also a lot of things are changing on their side it's not only we have quite dynamic situation on the technology end but it's also quite dynamic situation on business side and also just to keep also a regular contact to talk each other and try to understand each other it can help a lot from both sides and it should be like both direction it's not only data people come to business or only business people asking something from data it's mutual discussions and mutual conversations this is what we should strive to
63
00:53:02,080 --> 00:53:55,520
ALEXANDRA EBERT: more of. Fantastic, Anna thank you so much for taking the time it was incredible what you shared with us today very very much looking forward to the reactions we're going to get from our listeners thank you for being on the podcast. Thank you as well Alexandra. It was wonderful talking with Anna and getting all these perspectives on how to make data democratization happen at a large decentralized organization. I hope you found this conversation as worthwhile as I did. I definitely learned a lot from Anna. As always if you have feedback for us comments or concerns reach out to us at podcast at mostly.ai or simply by shooting us a message on LinkedIn. As always we will be back with an episode next week. Thank you for listening today.