Accelerating data science in finance and insurance with Jochen Papenbrock, NVIDIA
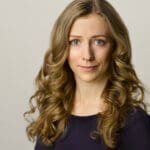
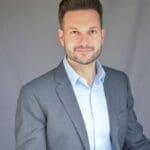
- How to implement AI successfully?
- How does NVIDIA help democratize AI?
- How to automate explainability?
- How is synthetic data used for evaluating and supervising AI models?
- What does the future of AI hold for financial institutions?
Transcript
Alexandra: Welcome to the Data Democratization Podcast. I’m Alexandra Ebert, MOSTLY AI’s chief trust officer, and here with me is Jeffrey Dobin, Duality Technologiy’s, privacy expert, and lawyer. Hi, Jeff.
Jeffrey: Hey, Alexandra. Good to be back. We have a very exciting episode coming up today. We have a guest from NVIDIA, and for those of you that aren’t familiar, they are pretty famous for their graphics cards and accelerated AI solutions and also their skyrocketing stock price.
Alexandra: Absolutely. Today I had the opportunity to talk to Jochen Papenbrock. A financial data scientist and financial technology manager at NVIDIA. NVIDIA is a global leader in AI computing platforms. Jochen in his work is helping large enterprises, especially financial institutions to leverage cutting-edge AI technology from first ideas to implementation. He’s also actively shaping the AI ecosystem and financial services by participating in public discussions and interdisciplinary projects. Really amazing what he’s doing.
Jeffrey: Sounds like he’s got a lot going on and I am certainly grateful he was able to make the time to speak with us today. Let’s go ahead and hear this interview.
Alexandra: Let’s do that.
Alexandra: Hello, Jochen, it’s so great to finally have you on the show today. Before we get started, can you share a little bit about your background with our listeners? Where do you come from? Also what’s your mission currently at NVIDIA?
Jochen: Thank you Alexandra for having me. Before I answer your question, let me highlight that these are my personal opinions here that I reflect and don’t necessarily reflect the opinions of NVIDIA.
Where do I come from? I am engaged in AI building, AI models in financial services, basically. Financial services means banks, insurance companies, asset wealth managers, but also recently the supervisors of financial institutions. The entire range. Because we see a lot of adoption of AI models in this area because it’s a very data-intensive industry.
Here in NVIDIA, we work on helping our clients to adopt these new technologies, to make best use of them using our computing platform to accelerate things, to make things easier, and really to go to production environments, because this is now the time to not just experiment, but really implement these.
Also, another part of the mission is to help customers to use some trustworthy AI and to manage the risks of using AI models because there are many benefits as we all know for using AI. But also we have to look at the downsides and the risks. We need a safe environment, controlled environment, and so we are compliant with AI regulation and governance.
Alexandra: Absolutely. I think it’s always about doing AI in a responsible way. Many of our listeners will know NVIDIA as this global gaming chips company, but there’s so much more to NVIDIA. Can you share a little bit about NVIDIA’s mission, also the business model behind it, and the role that you have both in the financial services industry, but also in your general AI ecosystem?
Jochen: Absolutely. NVIDIA is a very special and very interesting company with interesting business models, I think. Many people I talked to for the first time about NVIDIA still think it is a chip or gaming manufacturer for gaming facilities. It still is obviously, but it’s much more. We have seen that parallel computing and accelerated computing is emerging, and you can use these things in almost any industry in research, and this is what NVIDIA has done. Using the idea of parallelism to process large amount of data in parallel, basically, and to provide a hardware and software infrastructure to process large amounts of data in a very smart and also flexible way.
This leads us today to a company that is engaged in almost all industries and research ranging from autonomous driving to COVID research, health care, forecasting of weather, space travel, all these things. In financial services also we have established our pipeline for more than 15 years starting actually with Monte Carlo simulation because, obviously, high-performance computing platforms are really good at simulating data. Also not only data but processes in deep learning because that’s matrix multiplication. This is something that is embarrassingly parallel so we also make use of the computing platform here.
In the end, we are a company that is really good at visualizing things, virtualizing things to create high-performance computing environments and AI. Recently we also see applications where you combine these things. For example, you can construct or design an entire manufacturing plant, where you simulate the computers and robots, and you train them also and you visualize this entire landscape. Or let’s say you simulate a landscape and you train your autonomous car driving around millions of kilometers. You have really the intersect now between high-performance computing, visualization and AI. That’s what we see. By the way, we also see this in financial services recently, but I think we are going to talk about simulating data and AI in financial services in this talk later.
Alexandra: We definitely will, this will actually be one of my next question since you’ve seen really countless AI projects in the finance and insurance space. What are currently the top AI use cases for banks and insurance service providers? Can you share a little bit about that?
Jochen: In financial services, obviously, natural language processing is very dominant. It’s like a working horse. You can use it in many, many environments. Processing unstructured data like text is a real big area, and the use case are manyfold. Using it for risk profiling, for understanding financial markets, for trading, but also for compliance reasons and risk management reasons. Also obviously in banks, we have a problem with fraud still and anti-money laundering. A lot of money is lost for the banks, so you want to automate that too.
We see a lot of optimizations in trading space or in the IT. It’s really use cases we see for increasing the performance, reducing the risk or making the bank more compliant to things or serving the customer in a more specific individual way. These are basically the use cases. Also, we see a lot of use cases where we have a lot of interaction between employees in the bank using the AI. It’s these human-in-the-loop assisting properties that we see in the bank. NLP coming back to your question is really one of the most interesting areas.
Alexandra: Yes, I can imagine, and one thing that I would be curious to get your practitioner view on that since you mentioned it now is the time to really scale AI and not only experiment with it, but we know that still many enterprises are struggling with doing just that and really scaling AI capabilities. What’s the secret to AI success?
Jochen: The secret, I believe, and it’s actually not that secret, but [laughs] it’s using a number of recipes, a number of tools at the same time. In the end, AI implementation is really a very complex thing to do because you have involved data technology, business model, legal, customers, regulators. It’s really very complicated in the end, but we have all these tools that help us in processes. For example, a couple of years ago, it was really hard to implement such a machine learning model. You had some data engineers first and some data scientists, and then you needed to take care of deployment.
All these steps are really complicated. It’s a lot of steps and costly steps, and these days, things have become much more easier. With tools, pieces of software that help you, that are available for free for download in the open-source community but also in the NVIDIA NGC. That’s a repository where you can basically get pre-trained models which are checked, which can be used in transfer learning. There are deployment tools there, so you can really make your life much easier these days.
We sometimes talk about democratizing AI. Basically, you should really focus as a customer on the business model, on the implementation of this model, and how a data-driven or AI-driven business model or product really works to make it effective. Not caring so much about the hardware, the software, the stack, making life easier to really focus on the important things. This is something that the community has done a lot in recent years to bring these tools forward, this stack that you need for that.
NVIDIA also had a large contribution here, because as I mentioned at the beginning, we see ourselves as a computing platform company and that means there’s also a large ecosystem around that. Startups, software vendors, partners using our SDKs or software development kits, which are at the moment more than 150, and then more than 8000 startups in the Inception program and millions of developers actually using these tools. It’s an ecosystem that uses those tools and makes use of the software that’s already available. Don’t start from scratch but really benefit from platform companies like us, using also the open-source tools by the community. Yes, it has become much easier for all of us these days.
Alexandra: Providing the building blocks and not having to start from the very beginning I think it’s one point. With all these tools in place, what are the biggest challenges that you currently see? What are the roadblocks to really making AI project happen? What’s your advice to the financial services companies that you work with on how they can overcome those?
Jochen: It’s great to see that we are progressing here so well but also now comes the downside. As we see more and more, AI-based models or data-driven models in production, we see also the risk and the problems that emerge. Also, many financial institutions recognize that maybe this is a little bit tougher and harder, and more complex than they originally thought. We still see a lot of models fail in the end partially due to not using the tools that are available and not really knowing about these but also because AI models are a beast in their own. They need to be controlled, so they need to be explained, they need to be transparent.
We see a lot of activity here now in the past two, three, four years. There are also tools being developed that go beyond the MLOps tools, that really help you to basically screen and stress test AI models and validate and explain them. It is partially something that can be solved with also computational power and smart algorithms. Not all of it obviously. We see an industry emerging around this, we see regulation emerging around this.
Just look at the EU AI regulation draft recently with a big focus on the trustworthy explainable AI on transparency. Also, on tools that help you to store your data and model to really have a historical, let’s say, repository to really look at what you have done, what the model has done before. Also, directing this regulation towards experimentation, and sandboxing environments, which is also very important because as I said before AI models are a beast, they are complex. You cannot plan everything before, so you need partners, you need an ecosystem and you need this dialogue and you need this learning curve. It must be an iterative process basically.
Alexandra: Yes, absolutely, and you need to have this safe experimentation environment. Now you actually took like three of my questions away so let us go a little bit back because I really wanted to get your take on explainable AI. I know that this topic is dear to your heart. What is explainable AI for you and why should making algorithms explainable be high up on the agenda for every business engaging with AI?
Jochen: It’s a little bit of a dilemma. The more complex your model, the less you can understand what the model has learned. If it’s still working well or if there are strange things going on in your model, especially when you update your data and parameters. That means you need to have a loopback into the model somehow. One way to do this is to use approaches known as explainable AI or sometimes you will also find them as interpretable machine learning. Whatever you call them, it’s just something that helps you to understand your data better and it’s something that helps you understand your model better, and also the change of your model.
There are many approaches out there. Some people start to incorporate explainability from scratch per design. Other people, first of all, build an accurate model and later on take care of explainability issues. A post-hoc explainability approach. You can use visualization to visualize your data and also your model outcomes. We have a lot of tools and instruments, to get a better access to your model. You can still basically benefit from a more complex model that finds nonlinear relationships and at the same time not lose control. Explainability, by the way, is not the only one. In a responsible trustworthy AI, you look at many more factors, obviously, like unwanted bias, fairness.
Alexandra: Yes, fairness is definitely an issue, privacy is also an issue where we help quite a lot with our synthetic data. Coming back to explainability, is explainability always possible or which degree of explainability is actually necessary and explainable to whom? These are the questions that we oftentimes hear especially from some concerned AI practitioners that are afraid that something as powerful as machine learning that’s capable of doing things that human brains can’t comprehend, can’t be explained in easily human interpretable terms.
Jochen: There are no standards yet. There’s not something as explainable AI is solving all the problems. It is another layer of modeling. There’s this quote saying that all models are wrong but some are useful, so explainable AI is useful. In the end, you need to interpret the model and you need to know what can go wrong with this model. It’s just one building block, I guess, of many and they just help you get some more grip on your model, so that’s really important.
Talking about the audience of explainability, that’s a very good point because the audience of AI models is large and diverse. What do I mean by that? It could be that the internal data scientists just want to understand what the model has learned. Can they debug the model or improve the model? Or is it the manager of the business line or project or product that has to understand what the model has learned? Or is it the regulator outside of the bank, supervisors? Or is it the customer that has some right to understand what this machine has decided?
It’s very difficult to find the right language and the right level of abstraction of explainable AI. I think we are in a very good progress here but still, a lot of research has to be done. I’m really curious to see what are the next steps here. Explainability is something that also reduces the model a little bit because originally we wanted to employ AI, artificial intelligence to find connections, relationships that human beings maybe are not able or capable of finding. Maybe your model has found something really interesting, relevant and then comes the layer of explainability and explains to you what the model has learned.
Maybe the human beings supervising that is not capable of understanding what the model has learned. Sometimes we cannot really decide if it’s, let’s say, an error or something that the model has learned or if it’s really something that nobody has thought of. Think about this Go example. The grandmaster playing Go at first, he was like, “Ah, I will win this game because the computer is doing moves which are really strange, and I think I will win it.” In the end, he just understood post hoc that he was just about to be beaten by the machine. They are very inhuman moves. We have to learn this communication and feedback loop if it really makes sense. That’s the tough thing, I think, really to interact with the model, but we are on a good way. Many tools are being developed, but we have to be very clear on this, that there will not be a 100% solution. It will always be sort of a dilemma.
Alexandra: It’s always a balance that you have to strike. There are some AI models which are constantly updating themselves, constantly learning and how should humans even be able to keep up with all of these changes. Potentially we need some AI that understands the explanation of one AI and then maybe audits this.
Jochen: Yes, probably you are right. I believe that we can automate that to a certain extent, an AI checking another AI, and testing and the AI that at least gives some hints like in fraud detection, looking for a lot of fraud cases and then presenting to humans the most obvious ones and the most striking ones so the human can decide in the end, is this really something or not? It’s really like the last push of the button is made by a human. The second model, this controlling model or AI approach, can help to control things. I think there’s a lot of potential for automation and computing.
Alexandra: I can imagine. What would you say could be the role of synthetic data and explainable AI? How could it enable or support it?
Jochen: I would count it as one of these techniques for automation and for testing and validating other AI models. It is actually part of this layer that I was talking about. Synthetic data has the properties that you can design to a certain extent. You can arrange it in a way that it may be used to stress a third-party model. The AI model has to undergo stress tests with, let’s say strange and outlying data to really understand how is the model reacting because only in that case, or only by this technique you can understand what this model is actually doing. You cannot look inside the model but you can test with data input-output.
Also, synthetic data as I said before has many roles. Another important factor is the collaboration in such an ecosystem between, for example, startups and banks because AI models are complex to build and to maintain. It’s something where a community has to work together, experts from technology and banks working together. How should a bank really test startups that can help?
They need to set up a test environment and you need data for this. You need to produce synthetic data, for example, so you don’t have privacy issues. Synthetic data is a key component of this evaluating AI models, but it’s also a key component of collaboration in the ecosystem. My personal belief is that as we see strong growth of adoption of AI models, we will also see a strong adoption of synthetic data at the same pace, at the same speed.
Alexandra: I believe that as well because as you just said, it’s on the one hand in the auditing and regulation that you really can’t only look at the code of a model. You have to have some data to make it visible how it makes its decision and how it may be interactive. Some strange data points, outliers, or unusual examples get fed into the model. You also want to ensure that those are for example, not treated unfairly, which brings us to another topic where synthetic data can help with making more fair data sets and helping to eliminate bias.
Of course, you highlighted the collaboration and development aspect of AI models both getting access to data within an organization. But as you mentioned, also sharing it with external parties, startups, vendors, and so on and so forth. Maybe one of the things that would be interesting to mention is the Gaia-X initiative that you were working on in the financial services industry. Can you share a little bit about this project and what the purpose of it is?
Jochen: Sure, basically what we are doing there in one of these topics or workstreams is exactly that. We want to build a platform using compute power and smart algorithms to help us validate and certify AI models. To do this in a way that is really efficient so, in the end, we are able to validate models very often during the day. You mentioned it, the model updates, the model changes, there could be concept drift in the models.
There’s frequent rebalancing, retraining, and this has to be monitored and supervised. Also, there are many parties that would participate in this, many startups, many banks. We have a lot of AI models in the future to be validated during a business day. If we are able to design a platform that’s really effective in doing that, it helps the entire industry and the consumer in the end, because we produce more trustworthy AI and at the same time there are no roadblocks basically so the industry can profit from this. Again, synthetic data is a key ingredient here. In our Gaia-X project called Financial AI cluster, we focus exactly on that.
It’s located in the finance and insurance data space of Gaia-X. We already have around 40 to 50 members, larger corporations, startups, tech hubs, and also politics and governments helping us. Also, we have feedback by supervisors, which is extremely important, I think, like sandbox environments. Synthetic data is an important building block among many others. At the moment, we built around 12 use cases and demonstrators there, and they are all linked and connected because they are basically creating this platform because it’s like a platform where you test and screen, and monitor AI models from very different angles.
Synthetic data, for example, is used in our project to improve the collaboration between the stakeholders. They can exchange data and discuss the same complex data, for example, but also we stress the models. Introducing synthetic data and seeing in a standardized way how the models react. Third, we are able to improve the AI model itself by removing imbalanced data problems. It’s an ingredient also in this Gaia-X project. It’s called financial AI cluster because potentially it could extend or support another project called financial big data cluster, which is already on the way.
I’m also engaged in another project where you look at sharing data and using things like secure data sharing and federated learning. These are very important because here maybe several banks together, they collaborate in sharing data somehow, but not really exposing the original data to each other. By the way, synthetic data could also be a very important building block here. These are the topics that we see at the moment and we try to reflect them in these Gaia-X projects and to address them. This Gaia-X project is built on the legacy of another project that we were engaged in the EU Horizon 2020, a project called FinTech.
There we produced several use cases around explainable AI, trustworthy AI, using so-called Shapley values, which is one technique. We extended this in our Gaia-X project to two large datasets. Really you have the large datasets that are more realistic to the real ones in banks. There you see it’s not possible to run this on a small infrastructure. Whenever you think about algorithms and data, the third component, or at least the third, there are more is the computing infrastructure that you need to think about. Obviously a very hot topic at the moment, also in Gaia-X is the skills of the data scientists.
What skill set is needed to run all these things, to understand all these things. In the end, you need all these good data algorithm platforms and people who can run this and explain this.
Alexandra: That’s for sure as we have many of our clients are in the cloud quite likely also using some GPUs from NVIDIA. So accelerated computing and the necessary computing power is definitely a key ingredient in making all of this work. One other thing that would be interesting for me is, since you have the chance to talk with so many people from the financial services industry, would you say that trustworthy AI and explainable AI is really a high-priority topic for them? Did this change with the proposed new AI regulation in the EU, or was it a topic before that already before this regulation was proposed?
Jochen: That’s a good one. Well, to be honest, using complex models and risk managing complex models has always been one of the core areas where banks were competent in the past. Also, the regulation and the supervisory practice we see is quite similar. If there’s a bank running some credit portfolio and they have a risk scoring and then rating model, this needs to be discussed obviously and checked by the supervisor. Banks have done this before, running complex models and explaining them. Now we’re entering a new stage because the data amount is increasing, models are getting more complex, and we see more models in very different areas in the banks. All this just intensifies a lot. This is reflected in this regulation, but it’s not really a surprise to many banks really.
Alexandra: We felt it also with a conversation with prospects, that regulatory pressure started to increase already a few years ago. I think you also made a good point that trust is also something that was always super important for banks. Now AI might be a new technology, but ensuring that it’s trustworthy and they can still uphold the customer trust, at least from what I hear from conversations with banks, is still a topic of high priority for them.
Jochen: Yes, exactly. Everybody talking about the dramatic changes and disruption of the industry. Of course, that’s true, yes, but it still is around the same purpose. Also in the past, before we had technology, there was trust in doing financial transactions and the bank was an institution of trust. Now the bank gets more digital, uses more algorithms, more data, works more with FinTech companies, using FinTech themselves. It’s just very logical that the technology used also must be trustworthy. All these things are not really surprising. Surprising is the speed at which we travel and also the opportunities we have using AI.
Also, the research that’s underway, it’s amazing. People are very creative around the ecosystem. Using the right tools and infrastructure is key, and the right data, obviously too, because garbage in garbage out.
Alexandra: That’s always true. One thing that the listeners of our podcast always love is a good success story. Have you anything that you can share with us where the financial services organization successfully implemented an AI solution or really brought it into production?
Jochen: Sure. In my day-to-day work, I have always the same scenarios. We meet banks for example, and they want us to help them on this road to implementing AI. Most of the banks or some of the banks are able to implement these things themselves. They have a technology department, innovation department, they have data scientists, programmers. They have the infrastructure and we basically help them to use our computing platform. That means hardware plus the software which is even more important in an appropriate way, so they really make the best use out of this and really accelerate stuff. We are very well known for our records.
There’s, for example, MLPerf. It’s one of the official benchmark organizations basically where you have very practical use cases with large data sets and we hold most of them, in terms of performance. Our banks, our customers that we are partnering with, they make use of this acceleration. We can really help them to accelerate dramatically, especially for example, in the NLP space, the fraud detection space, the conversational AI robots, chatbots.
Also, recommender systems are the heart of the internet, or retail economy. Using accelerated AI for our customers is important not only because things are faster and real-time. That’s obviously good for conversational AI and fraud detection in real-time, but also to really make best use of the time of the data scientists. Really save that time so they can focus on the model building and not wait until the model is finished or the data is loaded.
Alexandra: Yes, sure. You really want to make sure that data scientists use the time as efficiently as possible.
Jochen: Right. It’s a very scarce resource and the data scientists are really driving this, and everybody, like data engineers too. Really making their lives easier and more accessible. This is basically our success story, how we help banks so they can run new products, new models that others maybe couldn’t before, or they couldn’t do this before. Also, the other scenario that we see when I talk to our banking customers is something like, “Okay, at the moment, maybe we don’t have the resources or we don’t want to build them up ourselves, so we want cooperation partners.”
In that case, we have a portfolio of several hundreds of software vendors and startups where we know that they are really expert at certain problem-solving stories. People for fraud detection, startups for synthetic data, startups for this and that, we really try to match them. In the end, the customer, the bank, gets a turnkey solution collaborating with some of the startups or the software vendors. That’s the other option, it’s as easy as that, make or buy. In both cases, we can help because we not only have this computing platform but also this ecosystem with startups and software vendors. This is how we try to be relevant to our customers.
Alexandra: Yes. I can imagine that you’re then a really valuable business partner for especially these big brands. In one of our earlier conversations, you also mentioned that you see that there’s this big sharing movement on the way, I assume, especially for the second type of organization that really wants to collaborate with vendors and startups to develop their AI.
Jochen: Exactly. We have this Inception program where we work with the startups and we help them. We don’t take equity or something, it’s more about letting them know about the options we have so they can test our platform, our infrastructure and can build their model on this. They can accelerate their business. We can help them to go international to meet larger banks and insurance companies. We really do this matching of solutions, really. This is how we are relevant to the entire ecosystem. As I mentioned, we have these more than 8,000 startups in our Inception program. Not all of them obviously in financial services, but a lot of them and it’s getting more.
It’s very interesting that we started so early to work with these technology talents because these people are really amazing. I love to work both with the large banks who have these really huge models, huge customer base. This is impressive. It’s also impressive to see what they are doing in their labs, in the research and development and innovation labs, and also what kind of great ideas startups have and how they implement this. We help them obviously to fulfill these dreams they have, and to realize them. Not all of them make it, but most of them.
Alexandra: That’s always the game. I can absolutely imagine that this is an exciting spot to be in, to have the opportunity to see both sides. What happens when you bring the startup into the company that has the infrastructure and the relevant data together. Really, really exciting. Before we come to the end of our episode, what could the future bring, in your opinion? What’s the future of AI? How will it change, especially financial services?
Jochen: That’s a good question. Obviously, we are on a good development path at the moment, so this almost dramatic development will go on. We will see more models, better utilization of data, more data, more synthetic data. Models being built in a more trustworthy and responsible way. We will also see more and more use cases where we didn’t even think about using AI as a helper or supporter. For example, one of the areas that’s really interesting is sustainable finance technology. Using data for better investments because that really could change something also in terms of making the planet a little bit safer or greener.
Trying to understand what is the impact of certain things in the environment. Geospatial AI, for example, satellite imagery, all these things. Also to better understand the reporting of companies and the supply chains really to see how are things being produced and backed by data and AI. We check this AI so we don’t have greenwashing that much. Also at the same time, and this goes hand-in-hand to increase efficiency also in building AI models, we need more efficient production of this in terms of energy.
Also, what we see is that the financial supervisors also tend to use more data and AI in their supervisory mandate and for policy. I think we have a good chance now to improve the entire financial ecosystem and landscape, make it more safe, more inclusive, and also cleaner.
Alexandra: Yes, more environmentally friendly. What are actually the lowest hanging fruits for making AI more energy efficient, is it on the hardware level or software level?
Jochen: It’s both. In terms of hardware, especially at NVIDIA, we have some contributions here in the top Green500 supercomputing rankings, we are very high. Today they announced another supercomputer being the greenest in Europe. On the hardware level, you can do a lot on the software level also improving the conversion of the training. You can do that also and doing AI model training more efficiently.
Also, third one option is running supercomputers, sharing basically the resource, make sure that the resource is 100% used all the time and that obviously the energy resources for that are greener. Obviously, you can also work on that. Maybe a bunch of startups sharing a supercomputer, really make better use of this. There are many approaches to make things more efficient. It’s how you run the model, it’s on the hardware, on the software. Interesting to see what will happen here in the next year, coming months, and years.
Alexandra: Absolutely, and yet another argument for organizations to move into the cloud not only for cost efficiency and access to computing power but also for environmental reasons. Since you can really ensure that the resources are always used and do not have your service center standing around.
Jochen: Yes, it depends on that, their colocation. Also, some people use the hybrid approach, though some banks, make use of on-prem installation, so they have their own cloud environment and run the baseload there. This base is always running, let’s say, and when they need more resources, the peak, they go to the cloud, buy the base, rent the peak. We see these hybrid approaches a lot and also pre-trained models, that’s also a very interesting aspect.
Not everybody has to do the same training again. You do it once on a supercomputer, let’s say, and then people can benefit and take the GPU style NLP model, for example, for natural language processing and then use transfer learning to adapt the last mile to their needs. That’s more of the software way.
Alexandra: Yes, absolutely, that also 100% makes sense. Of course, then it’s increasingly important that you make sure that these building block models are actually audited correctly and that you don’t have any biases in there and so on and so forth. If you can ensure all these and I think we touched upon many things where explainable AI and also fairness are currently researched and more and more implemented. I think it’s really a great way to accelerate AI adoption but also have things like energy efficiency and environmental impact in mind.
Jochen: Absolutely.
Alexandra: Before we really end, the last question is, what would you say is the future potential for synthetic data, especially in financial services but also in other areas?
Jochen: As I mentioned at the beginning, in many industries, we simulate data. We have these virtual environments, where you test and simulate AI models like a landscape where you train autonomous cars, for example. You can do the same in financial services. Creating a large-scale testing environment for AI models using synthetic data. It’s basically the digital twin of the real world that you can use to train and stress and enhance those models. It really has a good future. Maybe I have to correct my statement before that synthetic data growth is at the same pace as the AI model adoption. Maybe it’s even quicker and faster and getting more attention in the future.
Alexandra: I won’t complain about it.
Jochen: Also, we are working on that. MOSTLY AI is one of the partners in our GAIA-X project among other startups. Collaboratively, we work here on making better use of the idea of creating data synthetically.
Alexandra: Yes, we’re definitely excited to be part of all these projects that are currently going on and the use cases that are covered in this initiative. Jochen, thank you so much for everything that you shared today and for taking the time, it was, as always, a pleasure to talk with you. If you have any final remarks for our audience that you want to share, then please do so.
Jochen: Thank you for the opportunity to discuss with you, it was a pleasure and I’m excited about the future developments of our industry. Also, I’m especially excited, obviously, on the new developments that you make, MOSTLY AI. I’m really curious to see where your road is going. I think you are on a very good way.
Alexandra: Yes, thank you very much. Really big things are coming up that I can’t disclose now, but everybody is excited about. We will have big news to share soon. Thank you very much, Jochen.
Jochen: Was a pleasure.
Jeffrey: Wow, this conversation was definitely inspiring and it’s great to hear about high-impact projects that are accelerating innovation in a good way. Jochen is certainly one of those people who put a lot of work into that.
Alexandra: Absolutely, he is. I really enjoyed this conversation. Talking to Jochen is a bit like traveling to the future since he has such a good understanding of AI trends.
Jeffrey: Totally. Let’s pull together the most important points. Although, I would say that this conversation was truly valuable from beginning to end.
Alexandra: I think so too, but still, just to recap what we’ve heard, let’s do this.
Jeffrey: All right. Number one, top AI use cases that he highlighted in financial services include NLP, which is natural language processing. He talks about this being dominant and you can use it in many environments. Processing unstructured data is also a big area and includes numerous use cases like risk profiling, understanding financial markets, trading, compliance, and even risk management. He also brought up fraud and AML as important areas in finance as well.
Alexandra: Definitely. The goal of these use cases is to increase performance, reduce risks and increase compliance, or offer personalized services.
Jeffrey: He said something really important that I think business leaders and banks and financial institutions should really take to heart. Now is the time to scale AI and not just experiment with it.
Alexandra: Definitely. The secret to AI success is using recipes and tools at the same time. NVIDIA has a large ecosystem with lots of software vendors and tools making AI implementation much easier. AI modeling must be an iterative process and there is a learning curve.
Jeffrey: He mentioned that a lot of models fail due to not using tools that are readily available.
Alexandra: Definitely. Then there are also tools beyond the MLOps toolkit which are becoming available for screening and also for explainability. An entire industry and regulatory landscape is emerging around explainable AI. We need explainable AI, not only to interpret and to help us to better understand the data and to model but also to follow the changes of the model over time. Jochen mentioned that responsible AI also includes fairness and privacy.
Jeffrey: Definitely, hot topics here. An interesting thought is the audience for AI models is obviously large and diverse. He talks about how data scientists probably want to understand how to improve models and then managers have their own KPIs. Then regulators expect explainable AI. You also have customers who also need to understand the decisions that are impacting them. It’s difficult to find the right language that fits all audiences. If you can get that right, a lot can be achieved.
Alexandra: Definitely, but it’s a tricky balance. Next takeaway. We can automate explainability to a certain extent and AI can check another AI. In the end, it’s a human that makes the decision. We could always imagine it like in fraud detection where suspicious detections or transactions could be flagged and then a human can take a look. Only those where the pre-checking AI flagged something which could really help us to scale this even further and ensure explainability across the AI landscape.
We also talked about synthetic data in the context of explainability, which really is one of the techniques for automation and for testing and validating other AI models.
Jeffrey: He shared how synthetic data can be designed for stress testing AI with strange outlier data, to really understand how your model is reacting.
Alexandra: I think that’s super important and synthetic data has many roles. Collaboration is also something that’s very important. In an ecosystem where you have lots of players, for example, startups and banks, you need to cooperate and you need to share data. AI models are complex to build and to maintain. It’s something where a community of experts need to work together and this only works if you can share the data.
Jeffrey: He also talked about how banks really need to test startup vendors. Obviously to do these POCs and to test these new vendors with cutting-edge technology requires data to test them. Banks need to set up a test environment. He talked about how synthetic data can be great for that purpose, to really avoid a bunch of the privacy issues and hurdles.
Alexandra: Definitely, and just get the data there much faster. He also shared that synthetic data really is a key component for evaluating AI models. Synthetic data is a key component of the collaboration ecosystem. Jochen really expects that the synthetic data field will grow as fast or even faster as the AI space.
Jeffrey: The Gaia-X initiative is building a platform using computational power and smart algorithms to validate and certify AI algorithms in what they hope is an efficient way as an AI model frequently updates and changes. There could be this concept drift and there’s this frequent rebalancing and retraining that needs to be monitored and supervised. Synthetic data can be a key ingredient in model supervision.
Alexandra: Jochen, also shared how synthetic data could be used in this project for plenty of things. Synthetic data can facilitate the collaboration between the stakeholders so that they can effectively, quickly and safely share data with each other. He also highlighted that synthetic data can have a role in stress testing models and really seeing in a standardized way how a model would react.
He mentioned that synthetic data can improve AI models by solving data imbalance problems. Really plenty of application areas for synthetic data here. One other thing that I really liked was that banks have always been institutions of trust. It’s very logical that the technology that they use must be trustworthy as well. Therefore, also it’s a priority for them to also build trustworthy AI algorithms and ensure explainability, fairness, and privacy protection along the way.
Jeffrey: He talks about the future of AI, bringing more models, better utilization of data, more data in general, more synthetic data, improve trustworthiness, and more and more use cases where we probably didn’t see nor expect AI to be utilized. One example he gave is sustainable finance.
Alexandra: I really liked that one.
Jeffrey: Sustainable finance is pretty cool. It’s an area where AI will accomplish some great things. He talked about using data for better investments, trying to understand environmental impacts. He also mentioned using geospatial data and satellite images to fight greenwashing.
Alexandra: Yes. That was exciting. He also shared that to make AI more energy efficient you should look at both the hardware, but also at the software level. One thing that he mentioned is that pre-trained models can be more energy efficient. For example, in NLP using transfer learning, and only training the last mile of the model, but not starting from scratch all the time can really help us to save loads of energy.
Jeffrey: He mentioned in the future, he believes financial institutions will simulate financial environments with synthetic data to really test and enhance these AI models.
Alexandra: We’re very much looking forward to this future. Really insightful things that Jochen shared today.
Jeffrey: Yes. Looking forward to it too. I think that a world with AI could really be safer and more beneficial as long as ethical standards are maintained and enforced.
Alexandra: I think so too. Thank you to everybody who listened today for tuning in. If you can give us a thumbs up and subscribe to our episode and to our podcast, this would be a great help and highly appreciated. See you next time.