Democratizing AI? Not Without Data Intelligence & Synthetic Data—Ari Kaplan on the Biggest Roadblocks to Scaling AI
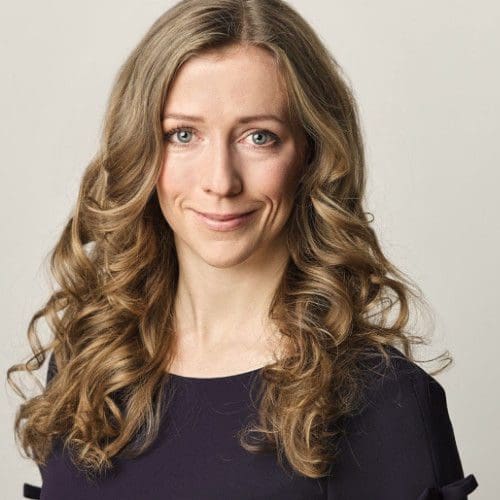
Transcript
1
00:00:00,000 --> 00:02:02,040
ALEXANDRA EBERT: Hello, and welcome back to the data democratization podcast. I'm Alexandra Ebert, your host and the Chief AI and Data Democratization Officer of MOSTLY AI. My guest today is nobody else than Ari Kaplan, the Head of Technical Evangelism at Databricks. And the conversation that we had, we covered a lot of ground. We talked about what drives Ari and the wow moments that he had in the past weeks and months when it comes to innovation in the AI space. Of course, I also asked him for a brief history lesson for our listeners in regards to the data lake house and the data intelligence platform. We talked about the top AI use cases for enterprise organizations, and particularly where he sees the strongest adoption for gen AI applications. And we spent a lot of time talking about the benefits of democratizing AI, democratizing data, synthetic data, and AI assistance that can help you to have conversations with your data and really getting value out of it, even if you're a non-technical person. But there's so much more in this episode that you will find interesting from Ari's secrets to publishing multiple books while traveling the world, how he approaches his social media content, and even the skills that he recommends employees of the modern workplace, but also his kids to acquire to be future proof in our fast moving world. So I'm confident that there is a lot in this conversation that you will benefit from. And with that said, let us dive right in. Welcome to the data democratization podcast, Ari. I was so much looking forward to having you on the show. And there's so much we want to talk about today. But before we dive into our conversation, could you briefly introduce yourself to our listeners and maybe share how the day of a tech evangelist looks like?
2
00:02:02,040 --> 00:03:09,520
ARI KAPLAN: Oh, and hey, great to be part of this. I love your podcast. I love your social media and also mostly AI. Happy to. But yeah, so I'm Ari Kaplan, head of what's called technical evangelism for Databricks. And the role of technical evangelism is an exciting one, where I get to travel around the world, speaking with different customers, partners, industry experts, what you might call influencers, and just hearing what are everyone doing with data and AI today? Like what are some of the incredible use cases that we see? But then also, what are some of the challenges? How can we, you know, raise ourselves? Like, we're all part of this generation now of making AI together. You know, the rules aren't fully set, the technology is evolving every week. So being part of that tech evangelism is like being part of the community, being out there and just trying to share any of the experiences that I see out there.
3
00:03:09,520 --> 00:03:32,440
ALEXANDRA EBERT: Fantastic. I think that's definitely one of the biggest perks, having the opportunity to talk with so many smart minds around the globe and getting the insights of where we actually are on our journey versus what is hype, what is future. And definitely very much looking forward to the insights you're going to share with us. One other question that I always ask my guests when they come on the show is, what drives you and what really makes you passionate to do the work that you do?
4
00:03:33,559 --> 00:04:20,760
ARI KAPLAN: Sure. Well, ever since I was a little kid, been completely fascinated with like, what's the newest, shiniest toy as a kid, literally. And then as you get to be an adult, you know, there's shiny new toys, but the toys become like incredible game changers, whether it's for enterprise business, which is really more where I'm focused, or, you know, the other passion is, how does technology, how is that helping shape, like, overall daily life? Like, just in San Francisco, and when in my first Waymo, like the first self-driving car, loved it. But that's what drives me is being at the drive of innovation, but not too far ahead, where you get an idea, but you can't actually enable that idea.
5
00:04:20,760 --> 00:04:22,760
ALEXANDRA EBERT: Execute on it. Yeah. That makes sense.
6
00:04:22,760 --> 00:04:43,880
ARI KAPLAN: Kind of like the bleeding edges, or the leading edge is what they call it. But that's like every week, every day, we're all bombarded by emails of this new launch, that new product. But that's what drives me. It's just so super exciting to be alive and in technology today, and then in data today, and then in AI today.
7
00:04:44,600 --> 00:04:53,640
ALEXANDRA EBERT: I can totally relate to that. So if you think back the past week, the past month, can you remember one of these wow moments where you were like, just mind blown of what's already possible today?
8
00:04:54,920 --> 00:06:57,239
ARI KAPLAN: All right, well, I'll do one Databricks one, and then one non-Databricks one. So we have been in private preview with this thing called Genie spaces. And, you know, every company, you know, that uses like business intelligence, it's great. But, you know, Genie is the ability, people just want to talk to their own data in their own language. So that's been very hard to do. You typically need to have some BI expert spending days, or weeks, or months writing a report. And, you know, for more complex reports, it's always going to still be the case. But if you could, number one, just talk to your data, and it understands the context of your information. And it has like the guardrails, where if you just ask, what does my co worker make in salary, if you don't have access to that information, you're confident that that will happen. So kind of gives you insights where traditional BI solutions don't, you know, it extends it, but then it also democratizes to non technical people. So that's one thing that loved and that's like in the last month, in fact, last night, some more capabilities dropped. And then off like I, I've been playing around with notebook LM, which is like one of the open things. So you're on a pod, we're on a podcast. Now, what I did is I took I co authored like a book, the data intelligence platform for dummies in PDF format, just uploaded it to this site, it understood the PDF, and it made a completely AI driven podcast with people going, Yeah, um, or fascinating, based on on the contents of the book. And it was actually really good. It had the intonations and people would love to hear that laughing. So that was awesome. And there, you know, things like that are dropping
9
00:06:57,239 --> 00:08:00,040
ALEXANDRA EBERT: every every couple weeks. Yeah, I'm so happy about the developments we see in that space. I'm personally I'm a dog owner, I love walking through nature. And with all these capabilities, you have nowadays to get articles read out and everything, it just allows you to work from basically anywhere in the world and in any setting. So I'm so excited about everything we see there. But coming back to the first point about genie spaces, I think that's such a fundamental puzzle piece when we think about AI and data democratization, that using your natural language not having to have this background of I don't know, six years PhD data science to be able to get value out of data, but just being somebody working in marketing sales, being able to interact with your proprietary organization and customer data and get value out of that. So I recently also was at money 2020. And at nearly every keynote, executives emphasize this importance and where they are on the journey. And I think these systems that Databricks and other organizations deliver just such an integral part of making that a reality. Yeah, no, it's um, first of all, like,
10
00:08:00,040 --> 00:08:56,119
ARI KAPLAN: can't wait to hear you if you have a recording on money 2020. That was incredible conference, I've seen, like some other presentations and social, but yeah, what a time to be all part of this. But like, talking to your data, you know, in the past, you need people who understand the data, understand like, there could be 10,000 columns in your, your entity relationship diagram or in your, you know, data warehouse. But they could all sound the same, like sales 059 sales 022. And you don't know what even what is a sale? Is it? Yeah, when you purchase it, is it 30 days past the return date? So just enabling people to have this like common, you know, semantic layer is great. Absolutely. And we know that. Exactly. We know that data assets
11
00:08:56,119 --> 00:10:57,320
ALEXANDRA EBERT: are growing and growing, but really understanding what the data tells you and figuring out what it is about, if you are not the person creating or collecting the data, I think there's so much potential for AI. And one other thing that we see, I mean, MOSTLY AI has been on this mission towards democratizing data access for over seven years now. And in the past, it was mainly this focus of unlocking customer data by creating synthetic data where you could overcome this challenge of privacy. But now combining that with AI assistance and seeing that business folks can now query their most sensitive data assets in a synthetic form is something that's just mind blowing. Because whenever you use just like more normally traditionally anonymized data, you always get reduced to the averages, you always get reduced to the average chain and John though, but with synthetic data, you can keep that granularity and cats get so much more insights into who your customers actually are. So we also had a conversation at Money 2020 with Databricks about a joint customer group, and they are actually the ones who have been using synthetic data ready since many, many years. And it just reminded me of that story where initially when they started out with synthetic data, they found some patterns about their customers that they wanted to develop a banking app, where they said, hey, that can't be real, there should be no human being having this type of income stream, this type of spending behavior, there must be a bug in the system. And this was in the very early days of MOSTLY AI. So we checked everything, we said, no, everything looks fine from our side. And then they went through all these cumbersome procedures of looking at the real data to figure out, yes, indeed, there are people, there are customers behaving different from what your presumptions are. And now with synthetic data, they could access that. So I think this will just be a game changer, combining gen AI assistance and making data more available to really drive customer centricity and developing not only for the averages of your customer base, but really for this full spectrum of human diversity.
12
00:10:58,520 --> 00:11:21,559
ARI KAPLAN: For sure. And with the synthetic data, I see some industries are even more apt to use it. So FinServ, public sector, healthcare, telco, those are all industries that are very apt. But then anyone that is doing sales, credit card information, gaming industry.
13
00:11:22,520 --> 00:11:35,960
ALEXANDRA EBERT: And actually car mobility data is another big one where more and more interest comes for synthetic data, because we know it's just, you're quite unique when we just trace your phone or your car movements and with self driving cars and the digitalization we see there,
14
00:11:35,960 --> 00:12:45,159
ARI KAPLAN: it's also an interesting area. Great. And one thing to pull mostly in is, just so you know, and the listeners know, I actually have done hands-on, like I put my hands on the tool. I had like Major League Baseball data set and I just uploaded and wanted to play around. And one thing, there's like synthetic data and there's a lot of things out there, but it's not just synthetic data, it's making it match up to align with reality. So for example, if you have certain people with income level, you want the percentage of people at each income level to be reflected in the data. So just by having the synthetic data, like analyzed on real data, you start to like get meta insights on your data. So you can find outliers, you know, spectrum of people's heights. So when you make that synthetic data, it's not just anonymous, but you're also learning and it mimics like the patterns and the distributions, histograms and so on of real life. So I found that pretty important.
15
00:12:45,159 --> 00:14:00,039
ALEXANDRA EBERT: Exactly, exactly. So it's really about retaining these generalizable statistics, but down to a very granular level while protecting the personal secrets. So in a synthetic data set, I wouldn't be able to figure out what you ordered at Starbucks, but I would see that people, I don't know, the 0.2% of a customer base with that in that profile would exhibit X, Y, Z behavior. And this is something that I think is super interesting, not only for machine learning product development, but also when it comes to using all the different cloud tools that are available, where particularly the organizations you mentioned earlier, the heavily regulated one, financial services, insurance, healthcare, still have this reluctance to put non-synthetic data or production data in the cloud environment. So it's a really, really interesting field. But now that we already ventured into synthetic data, I actually wanted to start with a little bit of a history lesson, because I think for our listeners, it would be super interesting to also better understand why the lake house is a concept that's currently discussed with so many enterprise organizations and why we actually arrived at the stage where we say, hey, we need to have a combination of the benefits and the abilities of a data warehouse combined with a data lake. So could you educate us a little bit about this? How did we get there? Yeah, absolutely. This is like
16
00:14:01,080 --> 00:17:27,160
ARI KAPLAN: part of my heart and part of my essence. I'm almost done with a book called The Data Lakehouse for Dummies, that Wiley series. So this is all through my brain. And also the next iteration built on top of it is the data intelligence platform for dummies, which is already out. But the lake house started many years ago and it solved a couple of challenges where there are companies that had a data warehouse, which is largely structured data, transactional data. When I say structural, that's like numbers, letters, date fields, and largely historical data. So that's been like one set of tools, like think Oracle. I was with Oracle for most of my career. And then the world grew and now you have all sorts of unstructured data. You have social media, YouTube videos, PDFs, Word docs. And so that's more file based versus structured database. Those are data lakes. So it's a very different format. And what you do with it is different. You could do different types of machine learning algorithms against that type of data, traffic patterns, weather information. So that was a completely different set of software solutions to largely incompatible. You'd have duplicate data in different areas, two different governance and audit trails, two different logins. And then two became three, four or five when you start adding real time data streaming. Sounds incredibly efficient. Yeah. And so costs would you double your storage? It wouldn't scale. It just stopped being efficient. So the lake house, based on open source, like Delta Lake, which gets hundreds of millions of downloads a year, is just a unifying environment to unify all your storage and your compute and all the governance, democratize it, help all of your workflow from the raw data to ETL extract transform load to making notebooks and language against it. So that's the lake house platform. It's a unifying platform for the first time that enables you to do all that, but do it the three benefits. Usually you get to pick one or two and jettison the third, but it's less money since it's a totally different architecture on files. For example, Parquet, it's more scalable. So when I mentioned Oracle having worked there, it would scale to tens of billions of records. And we thought back in the day, there would never, ever, ever be a need. But now we're talking trillions of records are very common or LLMs with tens or hundreds of billions of parameters are common. So lake house scales to pretty much infinity, trillions of records with really fast return rates and it makes it simpler. So again, you usually have to pick one of those three and sacrifice the other. But now as a result, the lake house is used by over 75%
17
00:17:27,959 --> 00:17:41,560
ALEXANDRA EBERT: of all enterprises. So that's like- I wanted to say nearly everybody, but you can definitely put a number to that. So one can say that while the house at the lake is the dream of many people, the lake house is really what you want to have for all your data and AI dreams to come true.
18
00:17:42,520 --> 00:19:22,040
ARI KAPLAN: Yeah, for sure. And it's a fun play on the word, data lake and data warehouse is the lake house. So that's where the industry has been. And now that most companies have done it and gen AI is like the new capability coming out on the market and people want to be able to get more intelligence out of their data. Lake house basically is the plumbing to store it all. That there's new platform paradigm, the data intelligence platform, which kind of like with the synthetic data or with the gen AI genie discussion, you have more intelligence built into everything. So on the business intelligence side, if you want to do a report, what are sales kind of understands what you want and can make dashboards just based on your experience. And I love having humans in the loop. It's all reinforced by humans, giving the thumbs up, thumbs down on the results, typing in a better answer. But also the AI is embedded when I said everything, not just in the reporting, but when you do any IT work, how do you scale up or scale down or more quickly start up a warehouse like with a SQL serverless. AI is used to understand this is going to be a long process. I'm going to dedicate a lot or a little when you merge fuzzy data, like you might want to merge synthetic data in one set with real data and another data intelligence platforms helps with like the fuzzy logic to match everything.
19
00:19:22,040 --> 00:19:41,640
ALEXANDRA EBERT: So it really helps to augment many of the steps that are done manually and repetitive as well as discovering also what we discussed earlier. I think prior to hitting record, discovering the data assets, better understanding what you actually have within your proprietary data. So it's a really sensible development in addition to the Lakehouse.
20
00:19:43,000 --> 00:21:35,959
ARI KAPLAN: Yeah, for sure. And it gets more and more when you have a company with 10 tables and 100 columns, maybe you can keep it in your head. But companies today have thousands or tens of thousands of tables and hundreds of thousands of columns. And just knowing where your data assets are, and then also knowing not only who accessed your data, but the converse is, do you have assets that people are not used? Yeah. Like the Texas Rangers can publicly talk about it. They were the world champion until about a week ago when the Dodgers won, but they had collecting all these data assets, every player, how their pitching mechanics are. And it was just sitting there and like two or three people were even aware that they had this data, but they would see this data was being collected, but not used and very valuable. So they were able to like send a newsletter out internally or discuss it saying, hey, did you even know we have this mechanical data on our players in the Dominican Republic? No, I didn't. This is fascinating. So even using AI to see what people are not using, it's just the whole democratization, more people have data. And then the other key part is if you're not familiar with how like machine learning works, the more data sources that you have in a machine learning process, it's not a guarantee, but oftentimes that data makes the model more accurate. Like the predictions are more aligned with reality. Doesn't always happen, but for example, if you're able to have more assets, like your sales combined with weather, you're selling umbrellas and it's rainy that that's going to-
21
00:21:35,959 --> 00:22:42,599
ALEXANDRA EBERT: Allows you to time that better or when you think about predictive maintenance and so many other things where it could really benefit or insurance. We know from our insurance clients that there's always a lot of interest to also add contextual information, additional data sets to really assess the risk much, much better as opposed to all the methods. So definitely a lot to go, but also coming back to the rangers example, I think this brings us back to what a holistic endeavor this whole journey towards data and AI democratization is. Obviously you need the tech stack, you need to make the data available. You need to empower people to make use of the data, educate them that it's even available. And then obviously this entire cultural transformation to make sure that this really changes how an organization uses and embraces data. So quite a long way to go, but definitely fascinating to see what is happening here. But one other question stuck to my mind when you shared that not only the Data Intelligence Platform for Dummies book, which you already wrote next to several other books, but now you're working on your upcoming book. How do you actually manage to do that? Traveling the world, doing your busy job and then also publishing one book after the other? Do you have an army of AI assistants
22
00:22:42,599 --> 00:25:22,560
ARI KAPLAN: helping you with that or what's your secret? Well, the tough thing is when I write, I don't, I try not to use, I actually do not use AI to do the actual writing, but hopefully all of us, you could use it to say, did I miss anything? What are the 10 features of Unity Catalog? And I can look through them just to make sure I'm not missing out on anything, but not even a but, and AI now is good enough that it not only provides here the top 10 things, but here are links to the sources. So I find it very helpful to be able to click through to the articles, like in the past you do a Google search and it would, sometimes it would be helpful, but other times you'd have to read through all the actual articles to get precisely what you want. So AI at least helps me answer the questions first, get the article second, but yeah, all the writing do on my own. I do find it fascinating. I have a couple of kids in college and one in high school and they are actually now encouraging students to use Gen AI. Like one of my kids is in cybersecurity learning Python. And I would have thought maybe they look at it as cheating, but they're like, no, you can use co-pilots, code assistants to help you debug your code, since that's the reality. But you have to get the program working and know how to upload it to GitHub and all that fun stuff. You still need to learn all the basics. So I find that fascinating. But yeah. So I never gave the full answer. At the beginning, the evangelist, I do travel around the world, but there's so many other things that evangelists do. And by the way, there are many other roles that are evangelists. It's just my full-time job. So there's concepts of field CTOs that oftentimes talk strategy, which is a great interest of both of us. There are developer relations or dev rel people that help organize hackathons and talk with like the open source community. There are always field engineers and solution architects that speak at events. But yeah, from the tech evangelist, I spent a lot of time writing blogs, making video like demonstrations, being in the product, speaking with partners, you know, trying to write blogs. But social media is not, you know, not always easy to do. You know,
23
00:25:23,360 --> 00:25:33,439
ALEXANDRA EBERT: exactly. What's your approach to it? So how do you manage to regularly get something out of out on social media and also make sure that you create something that as it does with your
24
00:25:33,439 --> 00:26:35,440
ARI KAPLAN: channels resonates well with your audience? Well, that that's one of the things I, I find exciting, like, I love seeing as many views as I can get, but also as much engagement as I can get. So I like to instead of just be a salesperson type of approach, here's what I do. People get bored on the feed. Like when I travel, I put personal photos as well as professional photos, and like, yeah, something, here's something about the local history or culture. But you know, tell it in a story that people could relate. Don't just state facts, read other social posts. So my mind, you know, we're all blasted with information, just to make it more interesting. You know, like, if you go to money 2020, don't just say the corporate line, but what was the experience like? Who did you meet? What was it like being on stage? So I guess the bottom line is to just be excited about what you do. And then hopefully that excitement comes out in your
25
00:26:35,440 --> 00:26:54,800
ALEXANDRA EBERT: writings and videos. That makes sense. That makes sense. I mean, we already talked a little bit about the data intelligence platform. But one question that still sticks to the back of my head is, what's your definition of when has an organization reached a point where they can say they use the data intelligently? Or what is data intelligence, if you would put it in a nutshell?
26
00:26:56,639 --> 00:28:46,160
ARI KAPLAN: Yeah, it's a, you know, general term, like, you know, the data intelligence is just defined, you know, insights that are actionable for your business. So in a way, you know, companies have always had data intelligence, it's, but now that you have a platform, an actual platform to help enable it, I think is the key difference. You know, being able to take all of your data and unify it together, and being able to more easily just ask questions, if you're a non technical person, you know, doing some natural language. And if you're a technical person, you know, it helps automate your boring, repetitive, time consuming parts of the job. And then you get elevated to do like the way more complex, or maybe you're doing probability to see, like, is this data in range, is this synthetic data, saying a human is zero feet tall, you know, that's, that's impossible. So that's, that's all, you know, part of the data intelligence, you know, platform is to just make that all as seamless as possible. And you know, there's, for every data scientist, there's about 30 business analysts. Yeah. So if you can get them to start asking questions, you know, answer questions that they had to go through technical people before, and you keep elevating everybody up, that's at least 30 times the value that you get out of your platform. And then the real value is in real life. If people are getting more informed, marketing people making more apt information to the public, salespeople saying the right messages, call center people being more efficient or more empathetic. That's really where the value takes place.
27
00:28:46,160 --> 00:29:30,320
ALEXANDRA EBERT: You're making wiser decisions. Absolutely. And also all these still untapped potential, we work a lot with financial services organizations, and everybody obviously, since years talks about personalization. And if you just look at this treasure trove of financial information and financial behavior, there's so much you can learn out of this data, who this person actually is, how they would benefit and really taking that information in and now with the technology we have available, also first getting this possibility to even do that would allow so much more in actually providing value to your customers and dealing with them on a much more differentiated approach as what is, what's commonly done with marketing sales and pro initiatives.
28
00:29:32,000 --> 00:29:47,519
ARI KAPLAN: Yeah, for sure. I love all those examples. And then everyone wins, the customer, the company themselves, the employees. It's incredible. And I can't wait to see where things will be going in a year or two, five, 20 from now.
29
00:29:47,519 --> 00:30:15,279
ALEXANDRA EBERT: Absolutely, absolutely. Since we talked quite a bit about the power of AI to now also empower people who are more data citizens than the data scientists, what are the skills that you think still need to be broadly distributed among our workforce? Or you mentioned your kids, what are the skills that you recommend your kids to focus on preparing them for a future where AI, AI agents can take over so many of the tasks that are currently still done by humans?
30
00:30:16,239 --> 00:32:51,760
ARI KAPLAN: Yeah, great topic that does hit close to home. You know, I have a kid who's a senior in high school and like, can I tell them, become a Python programmer? And that's your job. You know, I don't know if that's the future. So yeah, I look at it like the Venn diagram, there's like software programming, which I think still will be super important. But like the common, you know, you have the ability to write entry-level Python is all going to be automated. But the ability to write more and more complex programs is going to be, continue to be a valuable skill. So that's programming. Then math and statistics, I think will always be very important since you want to, you're going to get models and it'll say price your product like this, but it'll also tell you like, here's five alternatives. Here's the probability that this might happen. So you need that probability and statistics to make business decisions. And then you need, which my opinion is probably going to be the most important, people that understand the business, you know, whether it's your specific company, your bank, or the business, meaning you understand how humans interact with the bank, how the international markets work and people that know your data, know what information exists, what data sources there are. And for me, the most fun part of data science for me has always been feature engineering. So that's part of the business people. You have two pieces of data. The simple example is you have for a stock, the price and the earnings of the company and two separate things. And it may take a human, maybe you can automate simple ratios, but combined price per earning is even more predictive of a stock than those two separate. That's like the simplest example, but feature engineering takes people who know the business to come up with different ideas. You know, how should we price our products based on region, based on competitors, based on how close our competitive stores are to us, changing dynamics, the feds lower the interest rate. How does that affect? Should we change our pricing or should we keep it the same? So those three things, the business, people know business statistics and programming, but you know, we all have to be vigilant and keep our eyes on it since things are being automated so quickly. That's true. That's true. And I would also add
31
00:32:51,760 --> 00:33:42,559
ALEXANDRA EBERT: communication skills. And I'm also thinking at Casey Kosikoff from the former chief decision scientist from Google, which once shared, well, up until now, most organizations that claim to be data driven are more data inspired and decision makers tend to use data to make decisions they anyways wanted to do, but feel more comfortable about them. And really to become more data driven, it also needs this ability to know which question to ask, know how to avoid common biases, know how to actually change human behavior and get people to also accept data that maybe counteracts their former beliefs and something like that. So I think it will also be increasingly important to work on communication skills and also these decision skills that need to be kind of groomed when you
32
00:33:42,559 --> 00:34:48,000
ARI KAPLAN: work with data. You know, I'm a big fan of Cassie, had the privilege to speak on the same stage in Stockholm not too long ago, but yeah, she's up to great things and, you know, the topics, I 100% agree the communication, you know, we didn't even talk about ethics and countering bias, but, you know, how do you, you know, the theme of your data and your insights are only as good as how people implement them in the real world. So that is like the storytelling, the communication, positioning, timing of when you tell information, like in, I have a long background in sports analytics and you can come up with the most actionable items, but if the players don't do it, then it doesn't matter. There's actually a great book, it's getting old now, but it's still relevant called Big Data Baseball about the Pittsburgh Pirates, Clint Hurdle. We showed that if you were to physically stand here, you have an 80% chance of catching the ball, where you stand here, you
33
00:34:48,000 --> 00:34:58,000
ALEXANDRA EBERT: have a 10% chance. So for the people only having audio, Eric just pointed to the left and to the right side of the screen. So just for the people who don't see the video, sorry for interrupting,
34
00:34:58,000 --> 00:35:51,200
ARI KAPLAN: please continue. Oh, gotcha. Yeah, yeah. Yeah. Hi, everyone on audio. But yeah, so to the left or the right, you stand in one place, you have like an eight times chance of making it out, but that wasn't what they were grown up with. So it works, it saves about eight wins, eight or nine more games a year, but players didn't want to do it, even if their manager told them to. And it took months and months and months to finally get them to adapt. And then it became so prevalent that baseball just a few years ago enacted a rule, banning people from majorly moving, like from one part of the field to the other. But that's all part of communication. How do you get people in the real world to trust the data, trust the insights, and then take action upon it? Absolutely.
35
00:35:51,200 --> 00:36:25,599
ALEXANDRA EBERT: And I'm also thinking now many of the guests I have the pleasure of talking with on the podcasts emphasize how important it is for organizations to really succeed with data and AI at scale, that they manage to have this cultural transformation. And this oftentimes is inspired, of course, by the executives and by the top management leadership. So from your experience and the insights that you get in so many large organizations around the world, would you also agree that the most successful ones, the most forward looking ones really benefit from this tech savvy leadership? Or have you also seen examples where it was more driven from a bottom
36
00:36:25,599 --> 00:38:05,039
ARI KAPLAN: down side? Yeah, but it all depends. But I would say you're way more likely to be successful if you have top down leadership that has a culture that avoids groupthink, where you dictate, here's what we want to do. And we're going to make the employees fall in line to achieve this without question. But you want the culture where people can feel free to come up with better ways, different ideas, kind of percolate to the top. I'm not always saying a different idea is better. They're not always better. But you can at least have an environment where you try, have different ideas, can grow quickly. But if something fails, you know, you fail fast is the term as well. So top down. And I am seeing, like I posted on social media, so public companies like IF, like this incredible food delivery, Uber Eats like thing in Brazil, they have internal conferences, Procter & Gamble, Intel, they all have these top down conferences where they get different business units to come together and talk about what is our data estate look like now? What are we missing? What can we grow? What are other companies doing with data and AI? And where can we, you know, all grow from it? How do we transform maybe our BI team to know they're not losing their job from automation, but their job is going to become cooler. They're losing the boring parts of their job, but they're going to be able to make dashboards
37
00:38:05,039 --> 00:39:02,239
ALEXANDRA EBERT: that are more exciting. That's I think that's fundamentally important that you really get in these insights, these best practices, these lessons learned from not only different parts of the business, but also from outside or I also work a lot on this public sector and societal level of how can we democratize data? How can we prepare society for the stage and the generation AI? And I think this is also something that we definitely need for small and medium enterprises where there's so much potential to benefit from it. But particularly in Europe, there's actually quite little that is being done to spread the news and share the best practices of, I recently spoke at a conference and later on somebody having a funeral, a company or hairdresser, et cetera, approachment, how can I use AI? So I think this knowledge sharing is something that is very, very important to actually get more people within large enterprises, but also beyond benefiting from the tools that are already available. And that's what I love with the
38
00:39:02,239 --> 00:39:47,200
ARI KAPLAN: democratizations, like in the past, to implement like a massive, like database, like Oracle, when I was there, it would be hundreds of thousands, if not millions of dollars. So that kind of put certain things out of the reach of small, medium businesses. But now you could go to chat GPT or whatever and be equal in that regards to large companies. So a lot of those types of barriers, like the costs are dropping, the ability to get started is easier than ever, the ability to get started price-wise and also tech-wise. You could be that hairdresser company and start implementing insights on your Excel spreadsheets even.
39
00:39:47,200 --> 00:40:20,080
ALEXANDRA EBERT: For example, for example, and oftentimes also point people towards Ellie K. Miller, who I think does a fantastic job in really showing these different tools that are available, that can benefit so many people from all the different walks of life, different professions, and are quite easy to get success with. So I think there's a lot that we should do, many people are already doing great work, but we just need to double down on that to get more people to benefit from it. But coming back to the enterprises, what are the AI use cases, gen AI, non-gen AI that you are currently most excited about, or where you see the most excitement among the customers Databricks is working with?
40
00:40:21,200 --> 00:41:32,719
ARI KAPLAN: Yeah, well, every single industry, you can go through and come up with great examples. I was just in Boston at our Health Life Science Summit. And so that's one inspirational thing, the ability to better take care of patients, the ability to better deliver drugs from a supply chain standpoint, but also from a more targeted medical prescription perspective. A lot of times it was like a numbers game. You do chemotherapy, tax the whole body, even though it's a specific type of cancer. AI is very helpful to make more targeted immunotherapies and so on, like City of Hope in Pasadena, California, using AI to help with all of that. AI in operating rooms is incredible. So that's healthcare, since that helps lower costs, lower barriers, helps with the health of the world, quite literally.
41
00:41:33,280 --> 00:42:04,719
ALEXANDRA EBERT: Absolutely. And I think also moving us from treating illnesses to protecting the health span and the health of individuals. So we also know in medicine that still data is a little bit skewed or quite a lot skewed towards the male body. And now also with all these variables and the data that we collect there, I'm so excited to see where this will take medicine and healthcare over the next decade, as it just allows to not only have more equality in healthcare, but also figure out much more that helps us to actually prevent illnesses as opposed to just treating
42
00:42:04,719 --> 00:42:32,319
ARI KAPLAN: them better. You know, a hundred percent. And yeah, that bias in the data and the treatment, quite literally, you want it targeted. So there's none of those biases and the treatment is way better, male, female, age, genetic predispositions, which is incredible. Absolutely. Yeah. Healthcare, pick any industry, financial.
43
00:42:33,280 --> 00:42:46,800
ALEXANDRA EBERT: Since we have a lot of listeners from the finance industry, insurance industry, let's talk about those industries specifically. What are the use cases that you are most excited about or is he currently the most traction within Databricks customers from financial services insurance sector?
44
00:42:47,599 --> 00:43:53,839
ARI KAPLAN: Yeah. You know, everything from getting the better financial products to the right people, getting better predictions. So financial security, being able to give out loans at the more appropriate interest rates with the right risk factors. So that gets, you know, no matter where you are in the wealth spectrum, you know, you could hopefully get more micro loans at the lower end where companies were large insurance companies or financial companies were too risk averse. They know the risks involved. So that could extend further out for health insurance, you know, make things both more affordable for people, but also less risky for the insurance companies as well. So instead of just, and similar to retail, it's called hyper personalization. Yeah. You are getting the right products and prices and discounts to the right people, you know, at the right time. And so that's where everyone wins. Definitely. Definitely. Those are great use cases. Yeah. I would agree.
45
00:43:53,839 --> 00:44:05,920
ALEXANDRA EBERT: There's so many more. There's like... Of course, of course. I think we could continue four hours just listing down all the use cases. And when we talk specifically about Gen AI, what are the use cases that are currently implemented that get the most traction?
46
00:44:06,880 --> 00:45:13,119
ARI KAPLAN: Yeah. Well, right now companies, I would say the most traction are the chatbots for internal use. You know, companies are still a bit averse or a bit shy of having public, like do certain questions since, you know, whether it's hallucination, even if it's like one out of a thousand times, you know, you want to, depending on what you're doing, if you're like looking, you know, hey, you're a retailer, where are products in stock? You know, that's pretty low risk if it has some hallucinations. But if it's like, what's the price of a ticket? And it says the price is negative a thousand dollars, you know, we will pay you for a ticket. Like, you can't have that. So internal chatbots are taking off, like being able to have employees, you know, look through Slack messages, look through your own Salesforce or marketing data. Like, so that, you know, GenieSpace is like the predominant one, but, you know, really, since that enables it like in under 60 seconds for at least, you know, like a 5-10 table source, but that seems to be...
47
00:45:13,119 --> 00:45:27,680
ALEXANDRA EBERT: True. There's this video that you mentioned, maybe we can link it in the show notes where you demonstrate within 60 seconds how quickly you can actually do that. So if it gets published prior to us publishing the episode, I will definitely link that because I think it's a great example. But sorry for interrupting. Please continue.
48
00:45:28,239 --> 00:46:27,119
ARI KAPLAN: Yeah. Opportunity accepted. So yeah, under 60 seconds, I do a demo of how you, you know, if you already have the data, you know, it's like next, next, next, I select these eight tables, I'm going to call it Alexandra's health insurance GenieSpace. Perfect. And then you're done. And then, you know, you can tweak it by having AI define what the columns are, precede it with questions and answers. And that's it. Super easy now. So like these internal chatbots are, yeah, I think the big use case, but like there's so much like playing video games at night, toxicity detection. If you're playing like Call of Duty or Minecraft or things where you can speak to other strangers around the world, you can have it, you know, better match you with people that are exciting to play with, like strangers you're playing with around the world. Or it could detect and prevent, you know, people cursing or using profanity.
49
00:46:28,000 --> 00:46:42,400
ALEXANDRA EBERT: That's just mind blowing that this happens. And this, I think, also brings us back to the performance tech infrastructure that you need to have to really make this available in real time when you need it via this online platform. So, so cool that this is possible today.
50
00:46:43,199 --> 00:47:35,040
ARI KAPLAN: Yeah. Fraud alerts. I think being able, like call centers, I think are great. You know, the old paradigm is please hold, please wait, you know, press seven for this. Like the ability, when somebody calls, like it looks in Salesforce or some other CRM, it says, you know, this is Alexandra calling, you know, MOSTLY AI. Hey, they're a partner. They're on our marketplace. They've been a partner for, you know, whatever months, you know, here are the customers we have jointly. So like this type of information just pops up and gets updated, like in real time, that really helps sales people. It helps call centers. It helps marketing people. And it also helps the people that are on the, on the phone. You don't get frustrated. You don't have to explain your situation each time. And then it's also cost reductions because it's not
51
00:47:35,040 --> 00:48:23,599
ALEXANDRA EBERT: the call center agent having to dig up all of this information, but he can help people just much, much quicker. So this actually aligns with what I also hear from, from our customers that I don't really see Gen AI assistance being exposed directly to the customer just yet, but definitely access to internal knowledge being the Slack messages, the emails, et cetera. We know that documentation is something that oftentimes gets left behind. So we also know with synthetic text data, help them to unlock the privacy sensitive parts of that, to fine tune their own LLMs and make this knowledge available. But also, as you just said, using Gen AI in customer service interactions to just prepare answers or prepare information for the human being to augment their work and improve their quality. I think there's so many benefits that we can already see
52
00:48:23,599 --> 00:49:16,319
ARI KAPLAN: today. Yeah. Supply chain is awesome. Like so many things that are physical that are non but yeah, it's taking, you know, now taking anything that's structured, unstructured, putting it together, making sense, finding patterns that humans might not have noticed beforehand, detecting like drift and data. If you have a model that was art, like a predictive model that was already working and then the data changes, you know, new country has a trade partnership with another, the feds in America raise or lower the interest rates. You know, some economic thing happens, there's a flood in Spain, you know, that, you know, Gen AI can help synthesize all of that data in real time in ways that just wasn't feasible before.
53
00:49:16,319 --> 00:49:34,079
ALEXANDRA EBERT: Exactly, exactly. And this makes it much more actionable. As mentioned, I think we could continue talking about use cases for hours and hours. But since we don't have that much time left, let's maybe spend the last few minutes on talking about the future. So if you think, let's say, two years ahead, three years ahead, what are you most excited about?
54
00:49:35,680 --> 00:52:00,720
ARI KAPLAN: Oh, I, I love, I love automation. So I, when, ever since I was a kid, I would like try to find automation, like in the real world, like how can I get my door to open at the right time, just for fun. And then with programming, how can I automate and even make, for example, video games self-declare? How can I automate creating of poems? When I was in eighth grade, I won my school contest since I wrote a program to write Beatles songs in their style. So I've always loved automation. And I think that's going to continue and accelerate, you know, we're not on a linear path. Things are linear and then things jump up from it. So from enterprise, you know, I'm excited to see more and more companies adopt this data intelligence platform and get incredible use cases out of that. You know, the governance, the scale of data, the sharing of data, cross companies, which synthetic data is going to play a central part, synthetic and non-synthetic. How do you share your data cross company, privately, securely when you need to? Those are things in the next couple of years from a business perspective. Then from the non, like just general world, like Gen AI is great for producing content. Admittedly, it's largely based on what humans have done and they're just merging it in fun ways. But like already, my son, I visited him at UIUC, University of Illinois, Champaign-Urbana. And he was showing me they already have video games where it's Minecraft, but it's not Minecraft. AI as you play is making these worlds as you play. And so already we have video games that like get created as you play them. Now, imagine a movie like I love Curb Your Enthusiasm. You know, what if I want to say make a Curb Your Enthusiasm based on this podcast or based on my social media or based on, you know, whatever topic you want. It will create like fun video content, movie content, almost like choose your own adventure. That's true.
55
00:52:00,720 --> 00:52:46,020
ALEXANDRA EBERT: And it'll be good. It will be really good. Yeah, yeah. I'm actually super excited for that point in time where we have the interoperability between different systems. When for example, we think about Netflix shows and then different wearables, where it becomes much easier to collect data about how are users actually enjoying what happens right at that second versus just an overall, okay, did I like that movie or not? And then having this much more granular Intel to from scratch create new AI generated entertainment. And I'm super curious in which direction this will take us and if we will get like hyper perfect TV shows or if they will engineer in human imperfections, because this is something that humans relate to more and more intently and something like that. So exciting times ahead.
56
00:52:46,559 --> 00:53:02,360
ARI KAPLAN: Yeah. And it'll like right now we can only guess, but I know it will be incredibly entertaining. Maybe not for everyone, but for me, hopefully for many of our listeners, it's going to be a brave new world.
57
00:53:02,360 --> 00:53:18,399
ALEXANDRA EBERT: Let's hope that. Ari, thank you so much for spending the hour with us. It was incredibly insightful and fun talking with you. I think the listeners will take a lot away and let's maybe continue this conversation and have a reiteration in one year or two years time to see where society is at that point in time.
58
00:53:18,399 --> 00:53:33,679
ARI KAPLAN: Yeah. Alexandra, thank you so much. Appreciate being on. I love your other podcasts and thanks to the audience. Thank you.
59
00:53:33,679 --> 00:53:55,699
ALEXANDRA EBERT: See I didn't over promise. I really, really enjoyed talking to Ari and I can't wait to have him back on the podcast. As always, I'm curious to hear from you. If you have any questions, comments, concerns, or feedback overall, just reach out to me on LinkedIn or write us on podcast@mostly.ai. With that said, looking forward to having you tune in next time.